Inaugural Levine Career Development Professor Named
Research by ENG’s Kulis makes it easier to analyze Big Data
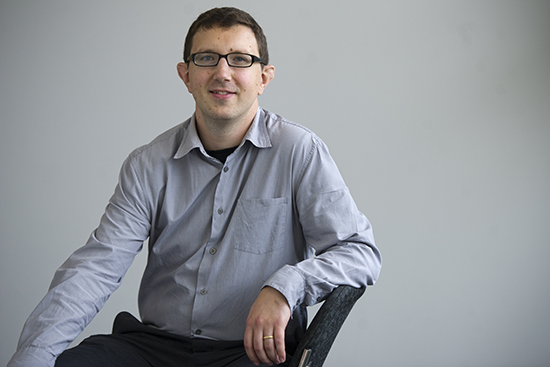
An expert in computer visualization applications, Brian Kulis, an ENG assistant professor of electrical and computer engineering, is the first Peter J. Levine Career Development Professor, awarded to rising junior faculty. Photo by Cydney Scott
To Brian Kulis, advances in machine learning and artificial intelligence bring with them the opportunity to mesh theory with real-world applications, like driverless cars and computers that can describe aloud the objects in front of them.
“You want computers to be able to recognize what they are seeing in images and video,” says Kulis, a College of Engineering assistant professor of electrical and computer engineering. “For instance, can it recognize all the objects in a picture? Or a more difficult problem would be, can it look at a video and describe in English what is happening in the video? That is a major application area for machine learning these days.”
Kulis’ expertise in machine learning, along with his research in computer vision systems and other applications, brought him to BU this fall and has earned him the University’s inaugural Peter J. Levine Career Development Professorship, which will be awarded annually to rising junior faculty in the electrical and computer engineering department. The professorship’s three-year stipend will support scholarly and laboratory work. It was established by a gift from Peter J. Levine (ENG’83), a partner at the Silicon Valley venture capital firm Andreessen Horowitz and a part-time faculty member at Stanford University’s Graduate School of Business.
Kulis is a rising star in the machine learning field and the Levine professorship speaks to BU’s recognition of his achievements thus far, says Kenneth R. Lutchen, dean of ENG, and is a commitment to helping Kulis build on his world-class research and teaching.
Lutchen adds that Kulis, who earlier this year also received a National Science Foundation Faculty Early Career Development (CAREER) Award for research into machine learning systems, will be a critical faculty member of ENG’s new master’s degree specialization in data analytics.
“We think it will be one of the most popular specializations we have, and it will be accessible not just to students in this department, but also to biomedical, mechanical engineering, and systems engineering students who will want to have this same specialization. Brian’s expertise is perfectly aligned with teaching this,” Lutchen says.
Also a College of Arts & Sciences assistant professor of computer science, Kulis earned a bachelor’s degree in computer science and mathematics at Cornell University and a doctorate in computer science at the University of Texas at Austin. He did postdoctoral work at the University of California, Berkeley, then spent three years on the faculty of Ohio State University before coming to BU.
Millions or billions of data points
Data science is about managing huge data sets—think millions or billions of data points, from an array of sources—and programming computers to analyze the data and make predictions based on identified patterns. Advances in storing and analyzing these growing collections of information has made Big Data a hot field in both academia and industry, with Harvard Business Review pronouncing data scientist “the sexiest job of the 21st century.”
Those advances include artificial intelligence and machine learning, and they are what enable Kulis to develop exciting connections between theory and action. “There is a nice combination between the mathematics and the theoretical aspects of machine learning. It’s a very applied field, trying to solve real problems,” he says. “That balance is pretty rare.”
He describes his specific area of research as scalable nonparametric machine learning. While a traditional statistical model for analyzing a large amount of data would establish a model for performing the analysis, Kulis pursues a different method. In his research, the data itself determines how simple or complicated the analysis should be.
An example of this approach, he says, is analyzing a large collection of documents for the content they contain. A parametric model would establish 10 clusters of documents to analyze, one each on a set topic. A nonparametric model would instead analyze all of the documents and determine how many topics should be included in the analysis. “You want the data itself to guide the discovery process, and so if there is a lot to say, then you want your algorithm to reveal that structure,” he says. “It’s a more flexible way to do analysis.”
The field is ripe for approaches that allow researchers from different fields—biology and business, for example—to apply machine learning techniques to develop new ways of looking at the data they collect. Kulis says he is looking forward to working with faculty and students from different BU departments both in research and in his courses. “Machine learning brings together a lot of fields that for a long time have been fairly disjoined. When it comes to teaching, a lot of my excitement is in trying to bridge these different disciplines and to teach courses that bring together people from different areas,” he says.
The curriculum, Lutchen says, has relevance to the world at large: “As an engineering faculty, we want people to understand how these new tools and techniques can help society.”
Michael S. Goldberg can be reached at michaelscottgoldberg@gmail.com.
Comments & Discussion
Boston University moderates comments to facilitate an informed, substantive, civil conversation. Abusive, profane, self-promotional, misleading, incoherent or off-topic comments will be rejected. Moderators are staffed during regular business hours (EST) and can only accept comments written in English. Statistics or facts must include a citation or a link to the citation.