Is There an Optimal Price for Group Rates?

By: Yunmei (Mabel) Bai, Doctoral Candidate, School of Hospitality and Tourism Management, Purdue University, and Chun-Hung (Hugo) Tang, Associate Professor, School of Hospitality and Tourism Management, Purdue University
Introduction
In the digital age, the focus of modern hospitality analytics, in both academia and in the industry, is identifying unique data to discover new variables that affect our businesses. However, understanding existing core theorems using current situations as assumptions and finding better solutions is also a crucial area in analytics that leaves much to be discovered within the hospitality field. The current working research presented in this article falls under this category of analytics which uses current situations that hotels face when providing group rates as assumptions to answer the question of whether an actual optimal price exists for group rates, as well as what factors affect it.
The Current Situation and Limitations of Group Rates
Current Group Business in the Lodging Industry
Hotel group business includes both leisure and business markets. Regarding these two different markets, group package tours are usually referred to as leisure groups (Moutinho et al. 2015) while business groups include national associations, corporate meetings, religious groups, and local social events (Kimes 1999). Group business also can be categorized into ad hoc groups and series groups (Yuen 2002). Series groups are usually repeat wholesale customers from tour operators (Guadix et al. 2010). They usually command deep discounts and high commission fees that erode hotel revenues. In contrast, ad hoc groups, non-repetitive group travelers, are considered a more profitable revenue source than series groups. However, ad hoc groups’ demand can be unpredictable and vary by markets.
Limitations of Current Pricing Practices
To answer the question of whether an optimal price exists for group rates in hotels, we first need to set the assumptions by finding limitations that hotels have when providing group rates. This study uses these assumptions to identify the possible group price boundaries that hotels can provide to groups. We start with the fact that group rates are set with a long lead time and cannot be easily changed, dynamically, as shown in figure 1, which is different from transient revenue management. At the time of setting group rates, hoteliers only have historical data instead of daily pick-up and reservation updates. Secondly, group pricing usually involves a bidding process and/or negotiation between sales personnel and event planners (Guadix et al. 2010). This means once the group rate is agreed upon, hotels cannot continuously update it in response to demand and supply changes. The process also suggests that individual guests’ preferences are superseded by event coordinators which means that each guest preference is considered less important than the collective good when group rates are applied. As for current group pricing that is being used, it is based on the displacement analysis by calculating the minimum acceptable group rate in a monopoly market. However, group rates are determined through a bidding process in which all interested hotels can only propose a one-time, fixed-rate nature (Farley 2003). The “one-time” chance to win in a bidding competition mandates the optimal group rate, not the minimum price. Finally, the group rates of competitors should also be considered, and the hotel should quote the most competitive, yet profitable, price, not simply the breakeven price.

Building an Optimal Group Pricing Model
We now move on to building a group pricing model to investigate how group prices are determined by both internal and external factors. Hotel room capacity is one of the internal factors which is shared by both transient demand and group demand (Yuen 2002). The optimal capacity allocation is determined based on the tradeoff between dilution and spoilage. Specifically, group rates are lower than transient rates (Guadix et al. 2010), and accepting too many group businesses could dilute the revenue by displacing high-paying transient customers who typically make room reservations later than groups. At the same time, because room inventory is perishable, hotels also need group businesses to minimize the spoilage risk (Yuen 2002). Therefore, a valid forecast of transient demand for the current year is another critical internal factor to consider in making group-pricing decisions. As discussed earlier, only the historical demand data is available at this stage and can be used as the proxy for transient demand forecast.
Group size is another important factor to be considered in group pricing decisions. It is a materialization of group demand, which shares the hotel capacity with transient demand. The most ideal group size is one that can perfectly supplement the transient demand such that full capacity can be realized without displacing transient customers. However, hotels cannot choose a group size, and can only passively accept or reject the group size given by the travel advisor. What hotels can do is change the group rate based on group sizes. Finally, Competition should be an external factor considered for group rate decisions. Group businesses provide a foundation for occupancy and are a main revenue source during low seasons (Farley 2003). Groups are expected to bring ancillary revenues from banquet, catering, and visual/audio rental. Therefore, it is realistic to assume a competitive setting for the group market. Based on the above discussion, we can assume that the group revenue can be maximized when the group rate is set at the highest level when it is the lowest among competitors. Figure 2 shows the factors that are included in the model to identify the optimal group rate.
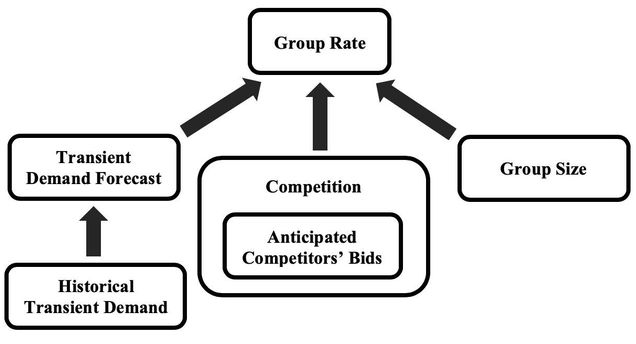
Using Visualization to Find Results
To better understand how the group rate can be affected by both internal and external factors, we run simulations and then draw plot graphs of the optimal bid price versus one factor at a time while holding other factors constant.
Figure 3 Figure 4 Figure 5
By completing this process, we discover several results about group rates. Figure 3 shows that there is a lower bound for optimal bid prices, which is indicated by the red box. The lower bound is the minimum acceptance rate when the group revenue equates the displaced transient revenue. In addition, the effect of the transient rate on the optimal bid price is contingent on transient demand. From figure 3, we can also observe that the transient rate begins to influence the optimal bid prices only when the transient demand exceeds 290 room nights. Figures 4 and 5 present the relationship between optimal bid price and the two drivers (transient rate and transient demand) respectively. The two slopes (denoted in red arrows in Figures 4 and 5) represent the sensitivity of transient demand or transient rate with respect to optimal bid prices. We can observe that the slope (Figure 5) of the transient demand forecast is steeper than the slope of the transient rates forecast (Figure 4) with respect to optimal bid prices. Therefore, we can further conclude that transient demand forecast has a stronger effect on optimal bid price than transient rates forecast do.
Conclusion
Analytics, broadly, is discovering and communicating patterns that we can identify using data or statistics. Using current limitations in group rates as assumptions and including variables that maximize revenue, this study runs a simulation that finds an optimal range of prices by identifying boundaries of group rates and group demand. More interestingly, we find that the transient group affects bidding prices after its demand exceeds a certain level, and the demand has a stronger effect on bid price than the rates suggested. This would suggest that practitioners would be able to maximize their revenue by concentrating more on demand than focusing on presenting higher rates to groups, which affects the bidding price.
Acknowledgments
We would like to give special thanks to Dr. Timothy Webb from Delaware University for the helpful comments. Articles accepted by Assistant Professor Sean Jung.
References