Distinguished Speaker Series: Fei Sha
Exploring Large Language Models for Reasoning and Language Understanding
Wednesday, March 29, 2023 | 10:00 AM
665 Commonwealth Avenue, Boston | Room 1750
BU Faculty of Computing & Data Sciences is proud to launch its Distinguished Speaker Series on March 29th with a lecture by Dr. Fei Sha entitled "Exploring Large Language Models for Reasoning and Language Understanding."
"We are delighted to have Dr. Sha share his insights on this very timely topic with our community" says CDS Associate Provost Azer Bestavros. "We look forward to welcoming him to BU and hearing more about his research."
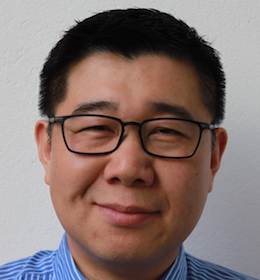
Currently a research scientist at Google, Dr. Sha is an expert in AI and machine learning who made significant contributions to the computing and data science field over the course of his career, focusing primarily on machine learning and its application to various AI problems: speech and language processing, computer vision, robotics and recently scientific computing, dynamical systems, weather forecast and climate modeling. Before joining Google, Dr. Sha was a full professor of Computer Science and the Zohrab A. Kaprielian Fellow in Engineering at the University of Southern California (USC).
Dr. Sha has have been recognized with numerous awards and accolades for his innovative work including being selected as a Alfred P. Sloan Research Fellow in 2013, and named an Army Research Office Young Investigator Award in 2012. He has a Ph.D. from Computer and Information Science from the University of Pennsylvania and B.Sc. and M.Sc. degrees from Southeast University (Nanjing, China).
Abstract
Large-scale language models have demonstrated amazing abilities in accomplishing many demanding tasks such as answering complex questions, composing articles and making slides, coding, or generating commands for robots. They are very successful in extracting knowledge from a huge amount of texts (as well as images, videos or sounds) and representing it in the model parameters. Despite the impressive performance, such models are quite easy to be "tricked", resulting in erroneous or undesired outputs.
In this talk, Dr. Sha will give a few such examples, focusing on two important issues: reasoning over factual information and interpreting linguistic expressions by combining their subunits (i.e., compositional generalizations). He will describe his research on tackling those challenges by exploring new learning methodologies. For the former case, Dr. Sha will describe his efforts in representing, storing, and accessing knowledge in a dedicated memory component. For the latter case, he will discuss various strategies to make learning models more “compositional”.
This talk is based on several joint works with Dr. Sha's former and current students, and collaborators at Google Research.