CDS Assistant Professor Joshua Peterson Merges AI and Behavioral Science
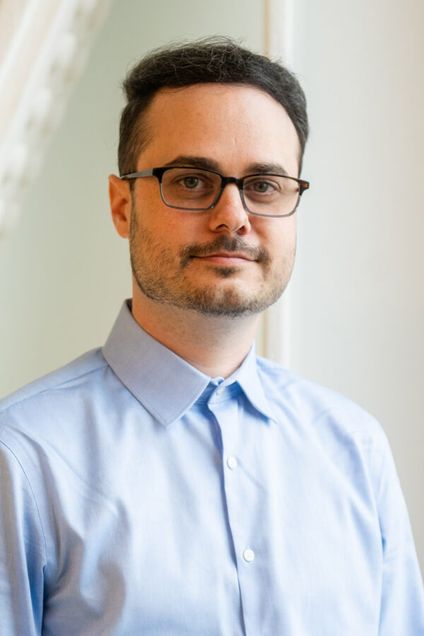
Photo by Cydney Scott for Boston University
Every day, we are faced with choices—what to eat, how to respond to a message, whether to invest in a stock. Although we like to think of ourselves as rational beings, making decisions by logically weighing pros and cons, the truth is often more complicated. Our actions are influenced by uncertainty, bias, and cognitive shortcuts. These limitations don't just affect individuals; they shape collective behaviors, economic trends, and the functioning of AI systems.
In an upcoming paper Large Language Models Assume People Are More Rational Than We Really Are, to be presented at the International Conference on Learning Representations (ICLR) 2025, Boston University CDS Assistant Professor Joshua Peterson and his colleagues examine how large language models (LLMs) represent human decision-making. Their study finds that LLMs tend to assume people behave more rationally than they actually do. For example, when predicting or simulating human choices, models such as GPT-4o align more closely with classical theories of rational decision making—how people should decide—rather than how people actually seem to make decisions in practice. Interestingly, the researchers also found that humans, too, tend to assume that others act rationally. As Peterson notes, “AI is increasingly being applied to interact with and even simulate people. An important open question is what implicit expectations AI has about human behavior. For example, do AI systems understand that human decision making can be irrational and subject to numerous biases?” His work explores not only whether AI can reflect human behavior, but also what it reveals about how both machines and people model the minds of others.
Modeling the Messiness: What AI Gets Wrong About Human Decisions
In the paper, Peterson and his collaborators set out to test how accurately today’s most advanced language models can predict human decision-making. They focused on two important experimental tasks from behavioral science. In one, participants faced choices between economic gambles, such as whether to take $100 with certainty or opt for a 50 percent chance of receiving $200. The AI models were asked to predict which option people would choose. In the second task, the models tried to infer what someone prefers based on observing their decisions. These experiments drew from over 13,000 human responses and were run across models like Open AI’s GPT-4o, Anthropic’s Claude 3 Opus, and Meta’s LLaMA-3. Across both tasks, the models consistently assumed that people would make choices according to rational, internally consistent strategies, such as always maximizing expected return or applying the same reasoning across similar situations. In contrast, the actual human data paints a more complicated picture. People often overweigh rare outcomes, exhibit a strong aversion to losses even when it is irrational, and adjust their decision strategies in response to subtle changes in context.
Interestingly, people also tended to assume that others would behave rationally. This shared expectation between AI systems and human observers assumes decision-making is logical and stable; however, this turns out to be false more often than we realize. In failing to anticipate human inconsistency, the models ended up replicating one of our own cognitive blind spots. Peterson explains, "The problem may not just be as simple as needing newer, better models. We found that larger, more powerful models that are more explicit in their reasoning—features that usually define good AI systems—have even stronger expectations of human rationality."
The Stakes of Misunderstanding Human Behavior
Peterson’s research highlights a growing concern: if AI systems are trained to reflect how people should reason, rather than how they actually do, they risk making predictions that are formally correct but functionally misaligned. This disconnect becomes especially problematic in real-world domains where uncertainty, emotion, and individual context shape behavior. Consider AI-powered recommendation systems for financial decisions or retirement planning. These tools, if built on large language models that assume human rationality, might consistently suggest mathematically optimal strategies, such as maximizing expected returns. However, as Peterson points out, “actual human investors often make decisions influenced by biases like loss aversion, recency bias, and emotional reactions to market volatility.” The result is a system that may offer sound financial advice, but one that people struggle to follow. When markets dip or uncertainty rises, individuals might panic-sell or abandon strategies not because they misunderstand the logic, but because their choices are shaped by emotional and psychological factors that the AI fails to model. These blind spots mirror the very gap Peterson’s study exposes: a persistent misalignment between how humans behave and how both AI systems and people expect others to behave. If models are to assist rather than mislead, they must incorporate not just idealized reasoning but the messiness of real human cognition.
Reframing Rationality
This research raises a deeper question: what happens when the systems we create to mirror us instead reveal our distortions? Large language models are often described as predictive engines, trained to reflect how people speak and act. But Peterson’s findings suggest that these models may also reflect how we wish others would think: rationally, coherently, and consistently, even though we often do not. In doing so, they expose a subtle truth: our assumptions about others are themselves a kind of model, full of blind spots and simplifications. Rather than seeing the failure of AI to capture human irrationality as a technical shortcoming, it may be more productive to view it as an opportunity—a sign to study the limits of human perspective as much as machine capability.
In reflecting on this idea, Peterson points out a paradox: “Modern AI models have seen more human data and read more psychology papers than any researcher, but they may not always fully leverage that insight to predict behavior.” Looking ahead, Peterson is interested in exploring fine-tuning methods that can help these models use their knowledge to help us in understanding ourselves.
Neeza Singh, Research & Communications Intern, Faculty of Computing & Data Sciences