Alex Matlock Wins Emil Wolf Outstanding Student Paper Prize
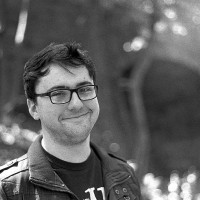
Matlock’s paper, “Physics-Embedded Deep Learning for Intensity Diffraction Tomography,” is recognized for innovation, research, and presentation excellence
At this year’s OSA Frontiers in Optics (FiO) Annual Meeting, CISE student affiliate and PhD candidate (ECE) Alex Matlock (ECE) won the prestigious Emil Wolf Outstanding Student Paper Prize for his work on “Physics-Embedded Deep Learning for Intensity Diffraction Tomography,” published in the September 2020 issue of OSA Frontier in Optics (FiO). This award recognizes the “innovation, research, and presentation excellence of students presenting their work during FiO.”
Matlock is a member of Professor Lei Tian’s Computational Imaging Systems Lab (CISL), and co-authored this paper with his advisor, Professor Lei Tian (ECE, BME). The paper devises a new label-free 3D computational microscopy method to quantitatively image biological objects in their native states without the use of additional contrast agents that would otherwise potentially alter the sample’s native behavior. The computational imaging field of quantitative phase imaging (QPI) has surged in recent years in an attempt to bypass this issue. QPI, through measuring light scattered from an unlabeled sample and computational reconstruction algorithms, can quantitatively recover a sample’s 3D structure and provide information about the biological sample’s local density, dry mass, and other underlying physical parameters.
Says Professor Tian, “Quantitative phase imaging has huge potentials in a wide range of applications, such as pathology, drug screening, and single-cell profiling. However, the adoption of existing techniques has been limited by the complexity of the instrumentation”.
CISL has recently developed an easily adoptable QPI technique known as Intensity Diffraction Tomography (IDT). The microscopy platform is built on an existing microscope modified by a programmable LED array. This system produces a lower cost, easier to implement, and more efficient 3D QPI technology. However, IDT’s simple physical model and computational efficiency is limited by its inability to produce high quality estimates for thick highly scattering tissue biopsies and large micro-organisms, as most tissue samples have complex structures that IDT would struggle to recover.
Matlock’s paper addresses this problem by melding IDT’s simple algorithms with physics-embedded deep learning for efficient and accurate complex object recovery. Using a rigorous physical model that simulate the IDT measurements under strong scattering, a deep neural network is taught to recover a high-quality prediction of a 3D object based on IDT’s initial poor-quality estimate. Once trained in simulation, this network was able to significantly improve the accuracy of experimental measurements on complex biological samples, such as cancer cells and C. Elegans worms.
“Because deep learning models can provide predictions in milliseconds, this research provides a new, fast approach to expanding IDT’s application range to complex biological specimens,” says Matlock. “Solving this hurdle will enable IDT to be easily accessible for any biological lab to implement and apply to researchers’ own work.”
Matlock is a 5th year graduate student. He was also a recipient of the NSF Graduate Research Fellowship. See more about Matlock’s work here.