POV: Navigating the Future of Higher Education: Learning Analytics Unveiled
Today’s education contexts have become more complex and dynamic than ever. What are the main factors influencing student success or failure in a course? How can we identify at-risk students and provide timely additional support? These questions are central as educators strive to develop a deeper understanding of learners and the vast possibilities of teaching and learning.
Given such, the field of learning analytics has emerged to offer systematic, labor-freeing, and timely solutions. This field measures, collects, analyzes and reports data about learners and their contexts to understand and optimize learning and the environments in which it occurs (Society for Learning Analytics Research).
Learning Analytics at BU
At Boston University, digital capacity is at the core of effective learning given the scale and diversity of its learning scenarios with 37,000+ students, 7000+ courses, and 300+ programs in various modalities. Our learning analytics leverage the wealth of data generated across the institution and sits at the intersection of 3 fields:
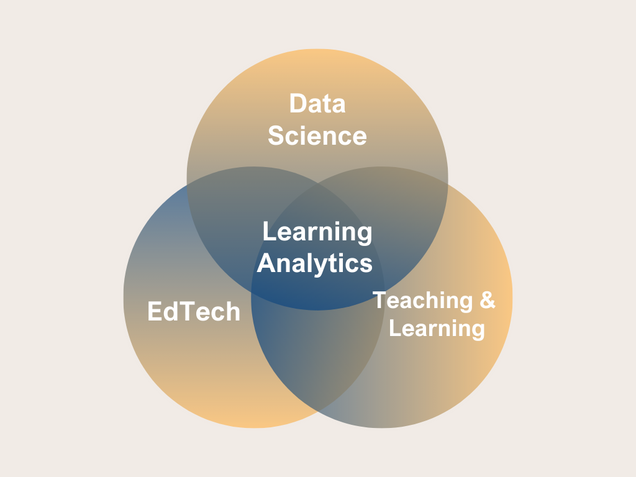
- Data science – Involves full cycles of data collection, analysis, and reporting of learning and learner data. Uses advanced statistical and machine learning models to analyze critical aspects of learning.
- Teaching and learning theories and practices – Serve as key references for asking the right questions and drawing interpretations of analytical results.
- Education technology – DL&I’s rich repository of edtech tools and services lays a solid foundation to generate data and allow analytics at scale to happen.
We closely follow the latest educational trends and incorporate advanced analytic techniques into our consultation and research. Here are some common use scenarios:
- Data collection: Educators may know what outcomes they are looking for but can have a hard time identifying what the right data points are or where to find them. We help instructors streamline data collection across edtech platforms.
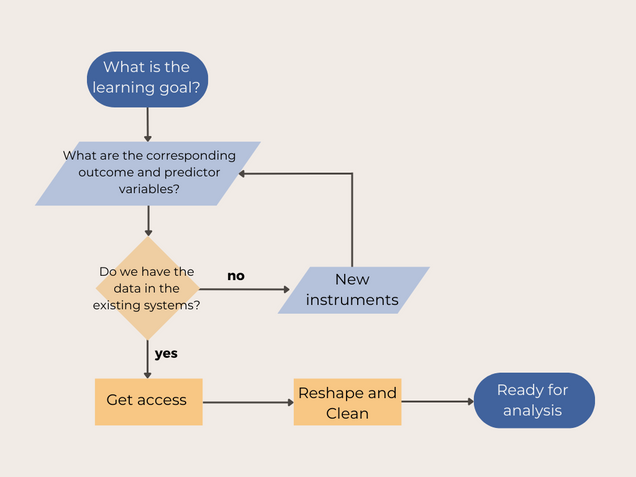
- Impact assessment and modeling: What are the impacts of education innovations on student learning? Learning analytics can draw inferences of the value-added by employing statistical models. We start with a thorough assessment of the learning scenario as the basis of choosing the best model for analysis.
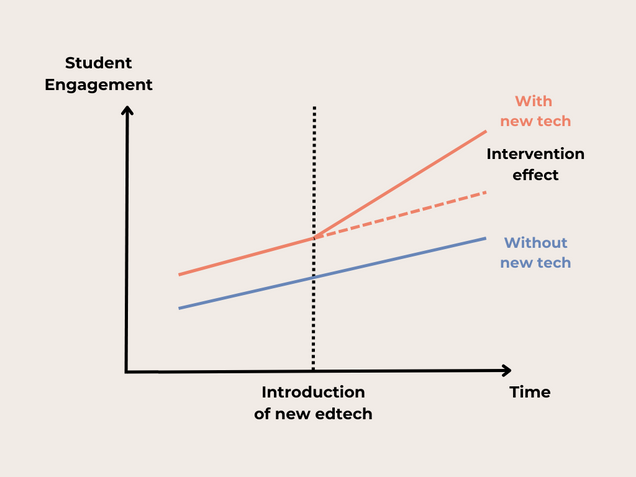
- AI and Machine Learning for prediction and pattern detection: To understand what factors can predict student performance or identify common patterns, machine learning algorithms are increasingly being used to analyze large datasets and offer tailored feedback.
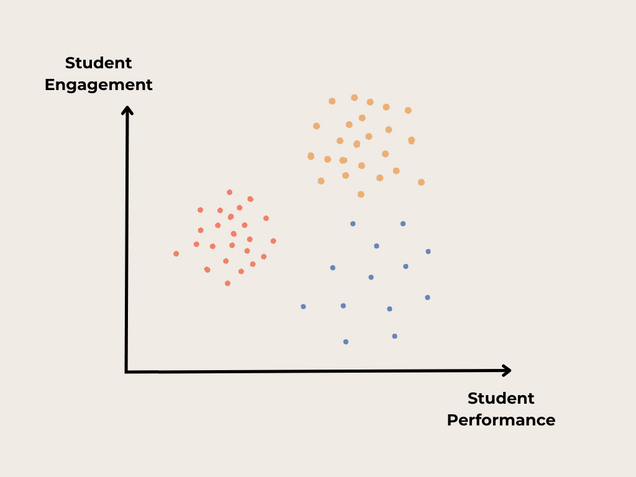
- Visualization and dashboard: By transforming complex data into accessible, actionable insights, these visual tools offer a clear representation of learning metrics to help educators pinpoint trends and tailor instruction more effectively.
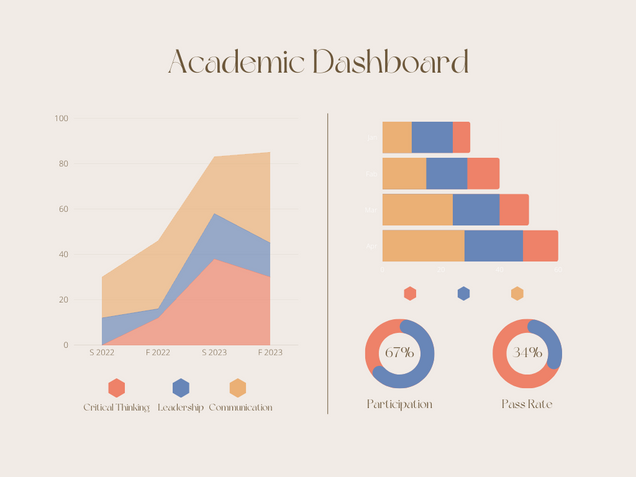
As we harness the power of data with learning analytics, we are offered a glimpse into the future of education where every student's journey is supported and guided with precision. The era of data-driven higher education is here, and its promise is both exciting and profound.
Interested in exploring learning analytics at BU?
Email Educational Technology for a one-on-one consultation at askedtech@bu.edu.
About the Author:
Shuang Geng (爽 耿) holds an Ed.M. in Learning Design, Innovation, and Technology from the Harvard Graduate School of Education and a B.A. in Statistics and Public Policy from the University of Virginia. She has previous professional backgrounds in policy research, institutional research, and assessment.