Adding Measurement Error to Location Data to Protect Subject Confidentiality While Allowing for Consistent Estimation of Exposure Effects
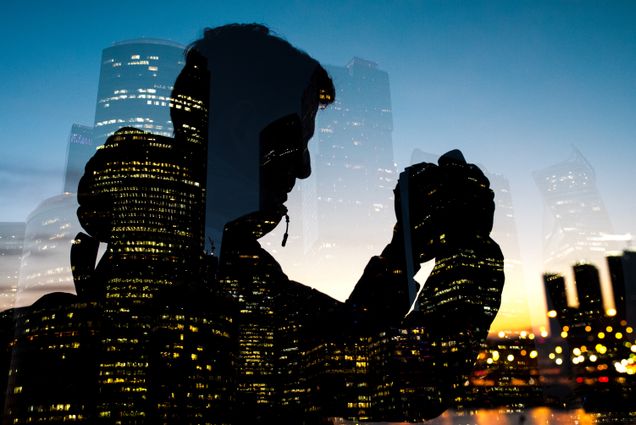
Many datasets involving human subjects contain sensitive information and require the protection of subject confidentiality. The protection of subject confidentiality can, however, limit access to the data, which in turn may limit the scope for valuable research. A common approach to addressing this issue is to create public use datasets that mask, or perturb, information that would directly identify subjects. Perturbation introduces noise to the dataset that can only be removed by authorized parties.
However, perturbing reported data can skew estimates and calculations for researchers using the publicly available data. In a new journal article published by the Royal Statistical Society, HCI Associate Director Mahesh Karra and two coauthors address the issue of perturbing public data in a way that protects subject confidentiality while enabling external researchers to conduct consistent and unbiased estimations. They propose an approach where a perturbation vector, consisting of a random distance at a random angle, is added to a respondent’s reported geographic coordinates.
Testing their method using data on perceived and actual distance to a health facility in Tanzania, the authors produce unbiased estimates with perturbed location data that are close to estimates obtained with the actual location data. Karra and coauthors acknowledge the success of their method depends on data perturbation that strikes a balance between transparency, replicability and confidentiality.
Based on their findings, they recommend five steps researchers can take when perturbing location data for public use datasets:
- Make the perturbation process simple and replicable,
- Make transparent the methods that were used to generate and add the perturbations to location data,
- Ensure access to, or make available, a population density map for the population from which the sample is drawn,
- Make available a covariate intensity map for key covariates that were used in the perturbation process, particularly if the range of location perturbations is large, and
- Assess the trade-off between larger measurement error, and more masking of subjects, against the larger confidence intervals in resulting estimates.