Event Recap: Connecting Data Science and Sustainability
BY: NATALIE GOLD
Sustainable living has become even more important, as the effects of climate change are felt by people all over the world. Scientists are working to understand climate change, its effects, and what can be done to combat the crisis. Data science and computational tools play a major role in finding ways to make everyday life more sustainable, and sometimes figuring out how data science tools can, themselves, be made more sustainable.
On March 8, 2021, researchers from across Boston University (BU) were given the opportunity to share how they use computational tools to discover ways to make life more energy efficient in the second installment of the BU Knowledge Transfer Series. The series is a collaboration between the Hariri Institute for Computing and BU’s Institute for Sustainable Energy (ISE). The “Computing and Technology in Support of Sustainability” event featured four BU researchers who use data science and mathematics to discover more sustainable ways of life.

Manos Athanassoulis, Assistant Professor of Computer Science, spoke about designing an efficient data storage system that uses less energy than cloud computing systems. Cloud data storage systems have become popular over the years because of their ability to transfer data to a remote data center for storage from any computer. The problem is, these systems use high amounts of energy when transporting data. Athanassoulis’s team used robust tuning, tuning that maximizes performance of systems for worst-case scenarios, and found that data was transferred faster. This could mean less energy used in systems, creating a more efficient way of storing data.

Ayse Coskun, Professor of Electrical and Computer Engineering, described their work using applied machine learning to automatically manage performance and energy efficiency in large-scale computer systems. Computing tools are big consumers of energy. Coskun wanted to lower energy costs of the technology while still meeting performance demands. One way to control the energy consumption of these high performance computing systems (HPC) is resource management. Coskun used system network monitoring and application profiling to understand what the system was doing and how it was using networks. Using data analytics in this way resulted in better job allocation and improved energy efficiency, without sacrificing performance.
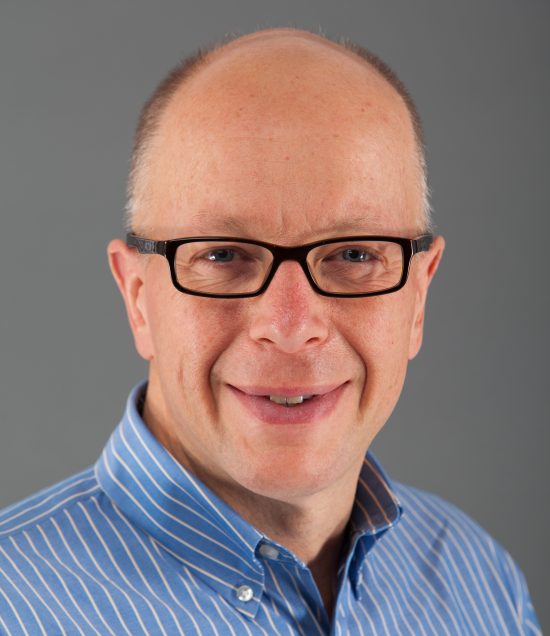
Mark Crovella, Professor of Computer Science, spoke about predicting where humans will move, and how this understanding could allow for better allocation of transportation resources. By analyzing people’s exploring and returning patterns, Crovella’s team categorized how people move: 1. scouters, who do a lot of exploration, 2. routiners, who tend to stay in the same place, and 3. regulars, who are somewhere between the two. Classifying people in this way allows researchers to more accurately understand people’s movement patterns. Crovella hopes this better understanding will make people rethink how they allocate resources for building transportation and possibly better schedule mass transit.
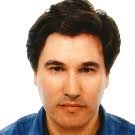
Julio Castrillon, Research Assistant Professor of Mathematics and Physics, explained an uncertainty quantification theory that ensures systems work in a timely manner and makes accurate predictions when faced with uncertain data. Uncertainty quantification is the science of quantifying and characterizing uncertainty in computational and real world systems. These quantifications address problems that come with incorporating real world variability into engineering and system analyses. Castrillon designed a framework so systems can operate efficiently when dealing with large quantities of data. Early applications suggest that the framework can decrease analysis times, possibly decreasing the energy use of systems.
The researchers took time after their presentations to discuss commonalities in their research and plan for future collaborations. Among the discussions, Professors Crovella and Castrillon spoke about future plans to use Castrillon’s uncertainty quantification work in the models on planning and predicting human movement. This mathematical framework could improve the time it takes to analyze some of the data Crovella uses.
There is much to discover about what can be done to address climate change, within both the data science world and the world at large. With the BU Knowledge Transfer series, the Hariri Institute and ISE gives researchers the opportunity to share their groundbreaking work, and discuss how to build on their research. This platform allows for greater collaboration in producing work that will impact the world.
The next part of this series, “Coupled Human-Natural Systems and Behavior”, will be held on March 31.