Event Recap: Advancing Chemical and Materials Science through Machine Learning
BY: DEIRDRE SHAHAR
Machine learning is an application of artificial intelligence (AI) that provides systems with the ability to automatically learn and improve from experience without being programmed by humans.
With the expanding use of high throughput computations and experiments, chemical and materials scientists can use the developments in machine learning and data sciences to propel the next generation of energy, biomedical practices, electronic materials, and more.
The Hariri Institute for Computing, along with co-sponsors BU College of Engineering, BU College of Arts & Sciences, BU Department of Materials Science & Engineering, and BU Department of Chemistry, hosted a symposium on Monday, June 14, 2021, to share some ways researchers have advanced chemical and materials science through machine learning. The event consisted of three sessions of key speakers from various backgrounds of science sharing their research, both individually and together as a panel and a student poster session.
The event’s first session focused on learning ways to generate data for chemical reactions that can be used for optimizing and automating reactions. These developments allow researchers to produce high-quality data that can be applied to algorithms used for their studies and experiments. However, a major challenge of generating useful data for machine learning applications is capturing the very large number of discrete and continuous variables that individually can dictate the reaction outcomes.
“There’s not one single thing that’s going to allow you to solve all of chemistry. You’re going to need to have multiple different types of instruments and approaches and utilize them where they best fit and with what you have available,” said Kerry Gilmore, an Assistant Professor in the Department of Chemistry at the University of Connecticut.
Speakers Connor Coley, a Henri Slezynger Career Development Assistant Professor in Chemical Engineering at MIT, and Grace Russell, a Scientist at Snapdragon Chemistry Inc., spoke about developments in performing organic reactions with laboratory robots and how to facilitate these chemical reactions through standardization and algorithms.
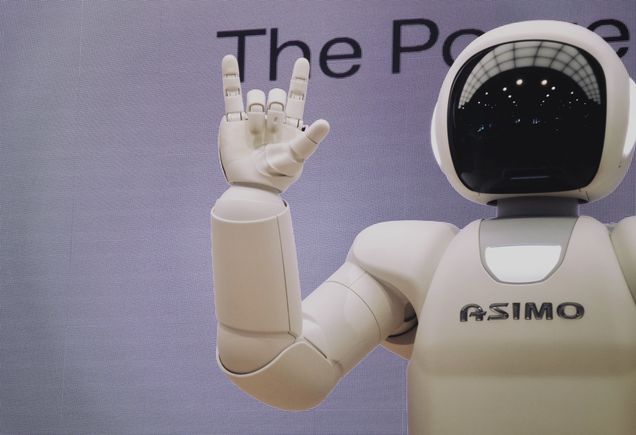
The second session of the symposium centered on some of the basic machine learning techniques and algorithms that are used for applications in chemistry and material science. Presenters discussed tools they have used to work with
structured data, such as networks of molecules. It is critical and challenging to map molecular structures while adhering to physical laws and providing accurate electronic structures in a way that can be used for machine learning applications.
Speakers Frank Noe, a Professor in the Department of Mathematics and Computer Science at Freie Universität Berlin, Risi Kondor, an Associate Professor in the Departments of Computer Science and Statistics at The University of Chicago, and Bidisha Samanta, a Research Engineer at Google, spoke about how deep machine learning methods have a profound impact on industry and information technology. They also spoke about the benefits of deep generative models for molecular graphs.
The symposium’s last session covered the promises and challenges of machine learning in computational chemical and materials science. Integrating machine learning and data science approaches with computational methods has the potential to allow more effective and efficient chemical and materials development. Data science is developing more flexible atomic-scale models, however, physics-based modeling is also beginning to inform data sciences. Leaders in the field discussed what direction this kind of research needs to go.
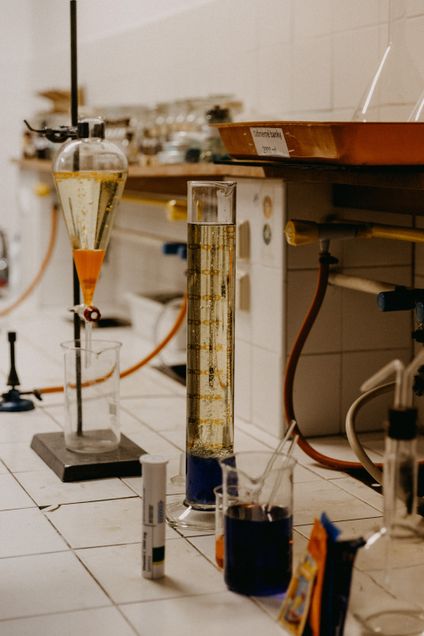
Pratyush Tiwary, an Assistant Professor in the Department of Chemistry & Biochemistry at the University of Maryland, showed that AI can also be used in chemistry to understand and predict the behavior of complex molecules with millions of atoms. He also explained AI’s several shortcomings, which Tiwary believes researchers can overcome through statistical mechanics. Yu-Shan Lin, an Associate Professor in Chemistry at Tufts University, and Michele Ceriotti, an Associate Professor in the Institute of Materials at Swiss
Federal Institute of Technology Lausanne, explained how researchers can predict the structure of cyclic peptides using molecular dynamics and how atomistic simulations in the age of machine learning have helped drive rapid progress in the field.
The symposium provided Hariri Institute Focused Research Program researchers the opportunity to connect with scientists from all different backgrounds on advancements in machine learning and chemical science.
“I think it’s very important to find where the interests match,” said Samanta, “and to work from there.” Chemists, physicists, computer scientists, and mathematicians alike have been working to develop new tools, techniques, and algorithms to further develop the use of AI. Kondor agrees that banding together with other scientists is key. “It’s important to build long-term collaborations to keep things objective,” he said, and that is something that the Hariri Institute hopes to achieve.
Want to learn more? Watch a recording of the event here.
Interested in learning more about the research happening at the Hariri Institute? Sign up for our newsletter here.