Ask the experts – What is algorithmic accountability?
BY GINA MANTICA
Artificial intelligence (AI) technology can determine your ability to buy or rent a house, gain employment, get approved for a loan, and more. But many of the algorithms that drive AI are biased and may lead to discriminatory business practices. Federal lawmakers introduced the Algorithmic Accountability Act of 2022 to Congress earlier this month to try to reduce inequalities in AI systems.
The bill requires companies that use AI technology to assess the risk of their algorithms, mitigate negative impacts, and submit reports to the Federal Trade Commission (FTC). The FTC would oversee enforcement and publish information about the algorithms that companies use to increase accountability and transparency.
We asked two experts from the Hariri Institute’s AI Research Initiative, Derry Wijaya and Bryan Plummer, Assistant Professors in the Department of Computer Science, about algorithmic accountability and whether or not laws can help mitigate AI bias.
What are some examples of algorithmic bias?
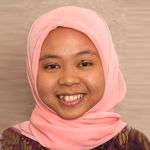
WIJAYA: Algorithmic bias is the systematic and repeatable decisions of computer systems that create unfair, discriminatory, or inequitable outcomes. Examples of algorithmic bias are algorithms that perpetuate negative stereotypes — for example, sentiment analysis systems that assign lower sentiment and more negative emotion to sentences with African American names, algorithms that have unintended consequences of censoring discussions about specific identity groups — for example, a toxicity detection algorithm that assigns comments containing mentions of disability as toxic, and algorithms that cause harms and potentially grave outcomes – for example, a facial recognition algorithm that mistakenly labels someone as criminal and revokes their driver’s license.
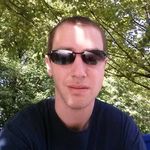
PLUMMER: Algorithmic bias is typically used to refer to an algorithm thinking a feature is important for making a decision when it shouldn’t be. A famous example is the Gender Shades work, where researchers analyzed commercially available machine learning models that would take an image of a person and try to predict their gender. What they found was that these models were biased towards predicting any photo of someone with darker skin as male regardless of their true gender.
What does algorithmic accountability mean to you?
WIJAYA: Algorithmic accountability means the process of holding some entities responsible or accountable in cases where the algorithms they develop or operate make decisions that result in unfair outcomes.
PLUMMER: I believe this puts the onus of whether your algorithm is unfairly biased towards some populations on those using the algorithm for their decision making. Making significant efforts to determine the efficacy and identify any unintended biases is a key part of algorithm development and becomes especially important for decisions that make a significant impact on a person’s life such as whether to give someone a job or mortgage.
Do you think that the Algorithmic Accountability Act of 2022 will reduce biases?
WIJAYA: Yes, I believe this is a good step in reducing algorithmic biases. A policy that sets up the mechanisms to examine and assess algorithms for biases will encourage efforts to reduce biases in the development and deployment of these models. In doing so, it will improve the transparency of these algorithms and hold developers or operators of the algorithms accountable for harm caused by biased predictions of their algorithms.
There are technical challenges including deciding what metrics are best used to assess algorithm bias and which part of the algorithms should be assessed in the case of a pipeline of algorithms. There are the practical challenges, such as whether it is the development or the deployment of these algorithms that should be assessed. There are also risks, such as whether or not this law will raise the barrier of entry for tech startups due to the cost of conducting meticulous assessments of algorithms’ potential biases.
PLUMMER: I am hopeful that any well-crafted bill could help provide the tools for those who are impacted by algorithmic bias to have some recourse to get their own ruling overturned, while also ensuring that others do not suffer the same fate. It will take a joint effort from all parties for this to be successful, but developing algorithms that ensure fair treatment is a worthy goal.
Should the government hold tech companies accountable for algorithmic bias?
WIJAYA: Mitigating harms caused by biased algorithms is an open research area in AI and there are increasing efforts within the AI communities to put some mechanisms to reduce biases. There is an effort to create “model cards” for published algorithms that document the parameters used to train the algorithms, the training data sources, the evaluation data sources, the intended use, and how the models perform across different situations. Having this be a rule of law supported and enforced by the government, instead of just grass-roots initiatives, would be very helpful.
*Responses have been edited and condensed for clarity.
To learn more about the Hariri Institute’s transformational research, click here to sign up for our newsletter.