Can an AI Model Learn the Changing Appearance of an Ear as a Baby Grows?
Betke and her team explore this question and win an IEEE IJCB award for their work
Before vaccines, millions of children died or endured life-long disabilities from diseases such as polio, diphtheria, measles, and meningitis. Unfortunately, there are still 20.5 million children who are missing lifesaving vaccines globally, 2.1 million more than in 2019, according to the World Health Organization.
Childhood immunizations are carefully timed to be given when the child’s immune system is ready. Oftentimes, identifying who has received a vaccine, what kind of vaccine, and when presents significant obstacles, particularly in developing countries.

Determining the identify of infants to facilitate vaccinations is important work being undertaken by Margrit Betke, a Boston University professor of computer science and co-director of the Hariri Institute AI Research Initiative. Along with her team of faculty and graduate student researchers from BU’s departments of computer science and global health, and the University of Zambia, she developed a method for identifying young children in Zambia using AI-assisted biometric authentication.
Biometric identifiers determine an individual’s identity through unique physical attributes, such as fingerprints and DNA. Betke’s team used the ear as a biometric identifier in a study involving infant children. The work, entitled “Age-constrained Ear Recognition: The EICZA Dataset and SASE Baseline Model,” won a best poster award at the IEEE International Joint Conference of Biometrics.
The research team’s objective was to develop an artificial intelligence model that could process the images of individual infants’ ears and create biometric identities for them. The motivation was to use such a model to enable re-identification of the babies for follow-up vaccinations.
“As people age, their ears often change in shape, size and characteristics, making the accuracy of biometric identification a challenge,” says Betke. “This is particularly true for infants and young children. So, we asked the question ‘Can an AI model learn how the appearance of a child’s ear changes as the child ages?’
To answer this question, the researchers first curated the publicly available dataset, “Ears of Infant Cohort in Zambia with Aging” (EICZA). The data set includes photographs of the ears of infants during their first year of life, taken by the team’s collaborators in a clinic in Lusaka, Zambia. Each infant was photographed multiple times from the age of six days to nine months, when ear growth is most significant.
The dataset, containing 3,330 ear images of 177 babies, is unique says Betke. “It is the first longitudinal dataset ever collected and involving young children. Before EICZA, the ear image datasets that AI researchers could use for their biometrics research include mostly adults without age information.”
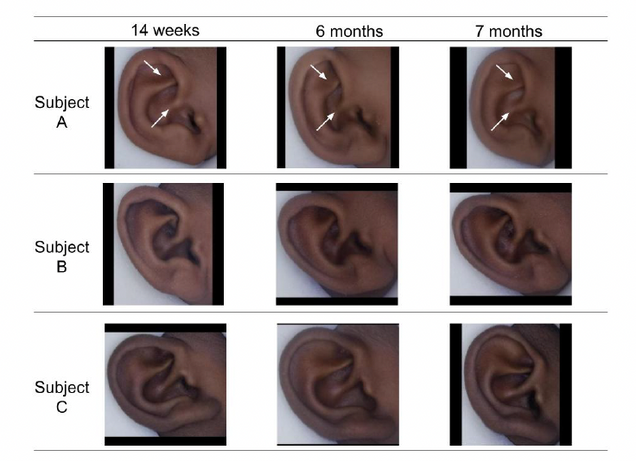
To allow recognition of an ear that had physically changed due to aging, the research team developed a new ear recognition model called Self-Attention-based Sequential Ear (SASE) image analysis. Rather than take a single image, the model takes a sequence of ear images at early but different ages as input. The model then processes these images learning feature representations that distinguish one baby from another. During inference, the model compares the feature representation of a query image with the previously learned representations that identify the infants.
The experimental results show that accounting for age allowed the proposed SASE model, when applied to EICZA, to outperform baseline models by large margins.
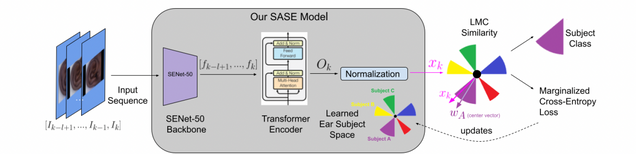
“This study showed the merits of the SASE model and suggests that accounting for age is an important criterion to consider in AI-assisted ear-based identity verification,” says Betke. “When trained and tested on the EICZA dataset, our current model’s accuracy is not at the level required for biometric identification of infants.”
Betke says that the next step in this work may be to enhance the data by adding images of subcutaneous features. “Subcutaneous images of adult palms, for example, are used by Amazon for their highly accurate biometric AI technology. I would stick with ears — infants have such tiny palms, difficult to photograph to yield images with biometric quality.“
Adds first author and BU Computer Science PhD student Wenda Qin, “Using the ear as a biometric identifier, particularly in healthcare settings for children, offers an important advantage over using the face – better privacy protection. “Requiring just a mobile phone to snap the image is also contactless compared to fingerprinting, which is increasingly valuable with the potential for pandemics.”
Learn more here: Wenda Qin, Lauren Etter, Alinani Simukanga, Christopher Gill, and Margrit Betke. Age-constrained Ear Recognition: The EICZA Dataset and SASE Baseline Model. Proceedings of the IEEE International Joint Conference on Biometrics ( IJCB 2023 ), Ljubljana, Slovenia, September 25-28, 2023. Best Poster Award.