AI-powered Breakthrough in Plasma Science Delivers New Computational Efficiencies
Unlocks Potential for Space and Fusion Research
Plasma physics plays a critical role in a wide array of scientific and technological fields, from understanding the Sun and other stars to predicting space weather, advancing fusion energy, and exploring cosmic phenomena like black holes and neutron stars. However, accurately simulating plasma behavior is computationally demanding due to the intricate interactions between charged particles and electromagnetic fields. Traditional kinetic models, such as Vlasov simulations, provide detailed insights but require immense computational resources, making large-scale or long-term simulations impractical.
Now, Boston University researchers have published a groundbreaking study in Proceedings of the National Academy of Sciences (PNAS) that leverages machine learning to dramatically reduce the computational cost of simulating complex plasma dynamics. This novel work in computational plasma physics was led by Dr. Ziyu Huang, Postdoctoral Associate at BU’s Center for Space Physics, under the guidance of his advisor, Chuanfei Dong, BU Assistant Professor of Astronomy and Electrical and Computer Engineering and Hariri Institute Faculty Affiliate, with contributions from Liang Wang, Senior Research Scientist, BU’s Center for Space Physics.
“This research focuses on nonlinear plasma phenomena, where plasma waves and charged particles interact in highly intricate ways,” explains Dong. “These processes have traditionally been difficult to model without expensive kinetic simulations.”
This research has broad implications for space physics, fusion energy research, and astrophysics. More accurate and cost-effective plasma simulations could improve space weather forecasting, aiding in the protection of satellites and power grids from solar storms. In fusion research, this model could enhance the understanding of plasma behavior in reactors, accelerating progress toward sustainable clean energy. Additionally, the ability to simulate complex plasma interactions more efficiently opens new possibilities for studying astrophysical environments, such as the dynamics of magnetized plasmas around black holes and neutron stars.
“By combining artificial intelligence with fundamental physics, this study marks a major step forward in computational plasma science, offering a powerful new tool for researchers across multiple disciplines,” says Dong.
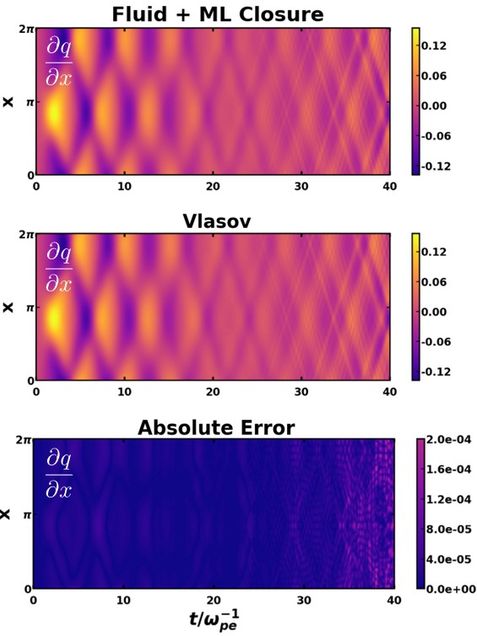
The breakthrough lies in the use of a machine-learning model called the Fourier Neural Operator (FNO), which efficiently integrates kinetic physics into a fluid model. Unlike conventional fluid models that often fail to capture nonlinear plasma behavior accurately, the FNO-based approach preserves essential kinetic effects while significantly reducing computational demands. The researchers demonstrate that their machine-learning-assisted fluid model can replicate the results of fully kinetic simulations at a fraction of the cost, making high-fidelity plasma modeling far more efficient and accessible.
The study was supported by NASA grant 80NSSC23K0908 and the Alfred P. Sloan Research Fellowship, with computational resources provided by the NASA High-End Computing Program and U.S. Department of Energy’s National Energy Research Scientific Computing Center, as well as the National Center for Atmospheric Research’s Computational and Information Systems Laboratory, sponsored by NSF.
To read the full study, visit PNAS here.