Shaking Things Up: How BU Researchers Are Driving Earthquake Understanding Through Artificial Intelligence
On January 27, the floors of New England houses, offices, and universities shook for about 20 seconds. Some thought it was a large truck driving outside. Others felt the tremors and knew something greater was at hand: a 3.8 magnitude earthquake off the coast of Maine.
Local social media pages and forums flooded with disbelief. An earthquake in New England? The Brink reports earthquakes around that magnitude only happen once a decade in this area. However, other areas, particularly those around the Pacific Ocean, experience them more frequently. Just this past December there was a 7.0 earthquake off the coast of California, and a 3.9 earthquake in Burbank on March 3. Most recently, a 7.7 earthquake caused significant damage to Myanmar’s second-largest city.
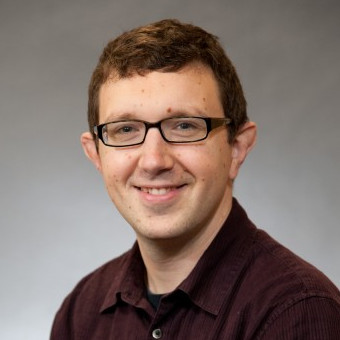
We can’t control when an earthquake happens, but could AI enhance the detection, monitoring, understanding, and early warnings of earthquakes? We met with Brian Kulis, Associate Professor of Engineering, (ECE, CS, SE), Core Faculty of Hariri Institute’s AI in Research Initiative, CISE Faculty Affiliate and Faculty of Computing and Data Sciences. Kulis shared his team’s findings in the Hariri Institute Focused Research Program (FRP) “AI for Understanding Earthquakes.”
It Starts with the Waves
Kulis has dedicated a large portion of his career working with machine learning and AI models. In addition to working at Boston University, Kulis also has experience as an Amazon Scholar in Alexa AI—creating AI models based on sound waves.
“In the music space, there are these core tasks,” said Kulis. “For example, if I give [the AI model] a piece of music, can it identify the genre? If I give it a piece of music, can it identify what key it’s in? If I give it a piece of music, can it identify the meter or the tempo? There are different problems that are considered kind of standard core music problems that a machine learning algorithm might want to tackle.”
As an expert in AI, Kulis has connected with other researchers within the Hariri Institute community to discuss the applications of artificial intelligence. During a conversation at the “AI for Understanding Earthquakes Workshop,” Kulis realized there was potential to apply his AI research in sound waves to seismic waves.
“Earthquakes are one of those fields where they do have people that look at using AI, but they’re not experts in AI, so a lot of the stuff they’re doing is on the simpler side,” said Kulis. “A lot of the techniques that they’re using are not the most recent kind of advanced techniques. I think there’s a lot of potential for people who work in the kinds of areas I do to back this research.”
Together, Kulis and his team applied for a Hariri Institute FRP to further uncover AI’s potential in the earthquake space. The FRP awarded them $100K and one year to pursue this convergent research in Hariri Institute’s community—leading to a $750k, three-year NSF grant, titled “A Large Foundational Model for Earthquake Understanding,”
Building Models
In collaboration with AI and earth science experts from Boston University, Los Alamos National Laboratory (LANL), and Harvard University, Kulis looked into current earthquake data to help build AI models.
The Parkfield Experiment seeks to understand the physics of earthquakes on the San Andreas fault. Since 1985, the experiment has gathered seismic, electromagnetic, and deformation data using sensors. Kulis and his team mostly used this data, along with the STEAD data set and data from Europe, to explore deep-learning architecture models, such as state-space models, audio encoders, time-series forecasting, and novel transformer-based language architectures.
“The models are doing a type of learning called self-supervised learning, where they take a little piece of the seismic data, a small window of time, and then they compress it into some very small representation,” said Kulis. “Then, the model tries to uncompress it back into the original seismic data. The model is basically learning to take a piece of seismic data and extract the most important information so that it can then reconstruct that seismic data from that little bit of information.”
The use of state-space models differentiates Kulis’ research from other existing research, as it’s the model he uses when analyzing sound waves. State-space models are time-series models and consider the current situation, how the situation can change, and what factors could cause change.
“For all of the problems that we’ve looked at [in music], state space models beat the state of the art, and it’s been surprising, because it hasn’t required a whole lot of algorithmic innovation,” said Kulis. “It just required applying these tools to these problems and seeing that they’re doing really, really well.”
The success of these models in analyzing data accurately could open the door to wider applications—such as gathering data from video surveillance cameras.
Expanding Research: Video Surveillance Cameras
With the development of AI models created specifically for earthquake detection and monitoring, Kulis’ team expanded their focus to explore other sources of data. This part of the FRP was led by Janusz Konrad, Professor of Electrical and Computer Engineering and Affiliate Faculty of the AI in Research Initiative at the Hariri Institute.
Some areas have Early Earthquake Warning Systems (EEWS)—systems designed to pick up on the earliest sign of an earthquake and alert the public. To understand these systems, it’s helpful to break down the anatomy of a seismogram into two parts: P waves and S waves.
The P waves are the first waves of an earthquake. They’re the fastest waves to travel to an area first. S waves follow the P waves. On a seismogram, the closer together the P waves are to the S waves, the closer the recording seismometer was to the earthquake.
EEWS are designed to notice these initial waves and magnitudes, and to alert areas that may be affected by the earthquake. California has seen success with these systems through a partnership with the USGS’s ShakeAlert EEWS. EEWS is operational and publicly available in all the west coast states at https://www.shakealert.org/.
However, other areas cannot afford the seismometer infrastructure for EEWS. Knowing a matter of seconds could save lives, Konrad and Prakash Ishwar, Professor of Electrical and Computer Engineering and Affiliate Faculty of the AI in Research Initiative at the Hariri Institute, uncovered another potential data source: video surveillance cameras. The key idea is that during an earthquake, cameras vibrate and these vibrations can be measured and used as a noisy proxy for seismometer data.
“Audio is essentially the same as seismic data,” said Kulis. “Seismic data is what the earthquake monitors are recording and that basically just looks like audio—it’s just a wave. Models for audio, it turns out, work very well on earthquake data.”
Using video footage, the researchers plan to extract vibration information and use it in their AI models. While the models may not be as reliable, the team hopes to position video surveillance as a potential alternative to EEWS.
Looking to the Future
By creating AI models that can accurately detect and monitor earthquakes, one can then hope to gain a better understanding of earthquakes. Rachel Abercrombie, Research Professor of Earth and Environment, is co-leading this portion of the FRP.
“There’s a lot of work in the seismic community about building synthetic earthquakes, like lab earthquakes,” said Kulis. “It’s not like a real earthquake, so people don’t necessarily understand how earthquakes really happen from those lab experiments. If we can somehow look at the data from the seismic readings, we might be able to learn something about the processes that are going on that are causing the earthquake.”
In addition to enhancing understanding about earthquakes, Kulis’ team seeks to improve the short-term prediction of earthquakes in addition to early warning.
“Can we predict if there’s another wave coming, and if so, can we predict how big an aftershock might be?” said Kulis. “There are various things that, even though we may not be predicting that there’s going to be an earthquake in California in a week, are very practical about prediction.”
Through the FRP program and the NSF grant, Kulis and his team will continue their research with a goal of sharing their findings on open-source platforms with the larger science community.
“The FRP program was extremely important in terms of getting this thing off the ground,” said Kulis. “And without it, I’m not sure any of these collaborations would have happened. We’re very grateful for this whole opportunity to be able to build this collaboration”
Kulis and his team will present this FRP at their symposium on May 8, 2025. Stay connected with Hariri Institute for more information.