How American Sign Language Helped Reveal Languages’ Hidden Patterns
Discoveries could transform how we learn, recover, and model language
Are words arbitrary?
For centuries, scientists believed that words are essentially random sounds that are given meaning. For example, there’s nothing about the sound of the word “dog” that tells you what it means—it’s just something we’ve learned. Following this logic, a dog could just as easily be called a “gleep” or a “zorbo”. Nothing about the sounds in “dog” make it a better label for our canine pals than “gleep” or “zorbo”. This assumption that words are arbitrary profoundly influences how we think about language, learning, and even how our minds work.
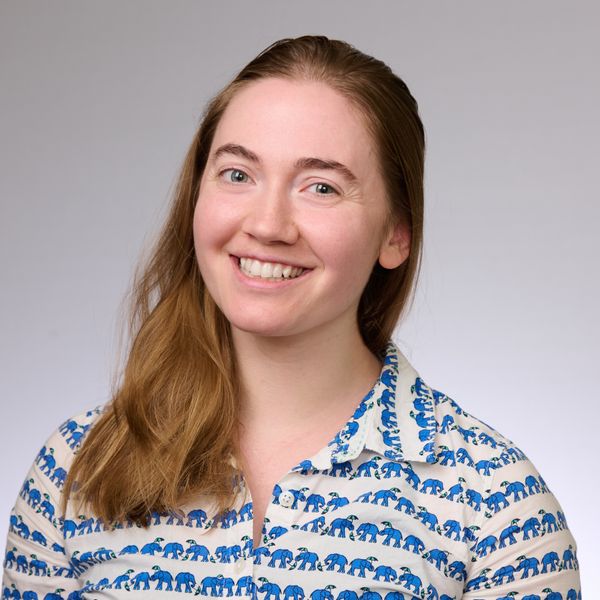
Now, a Boston University-led study has upended traditional thinking using an unexpected source: American Sign Language (ASL). The researchers found that in ASL—and in the spoken languages of English and Spanish—words and signs that mean similar things often look or sound similar. The findings suggest that words are not random; instead, their appearance and sound reflect meaningful, non-arbitrary patterns.
The findings, published in The Proceedings of the National Academy of Sciences (PNAS), reveal novel insights into how human languages work, opening new avenues for how we teach language and build future technologies.
Discoveries: Structure, Not Chance, Shapes Language
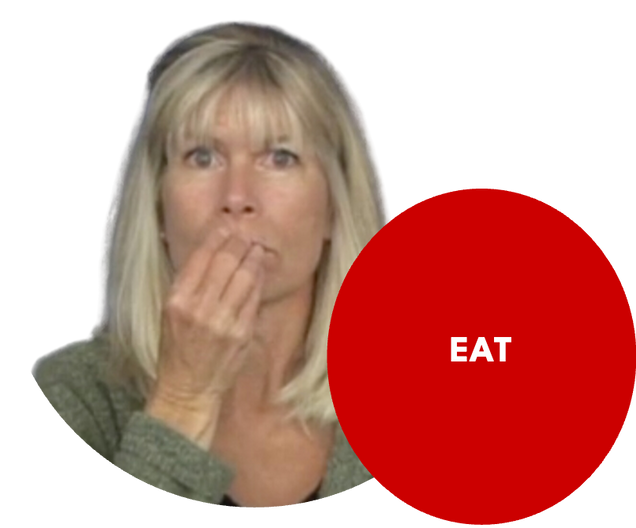
Languages (like English, Spanish, and ASL) have clusters of words that have comparable meanings and are pronounced similarly. Think about how the English words “glitter”, “glimmer”, and “glisten” all evoke a sense of light or sparkle, and have a similar phonetic quality. Researchers have known about these clusters, but considered them rare cases. One of the missing pieces has been understanding how these clusters (called ‘systematicity’) form.
A central discovery of the paper is that systematicity is far more common than previously thought. The researchers observed that 54% of ASL signs, 39% of English words, and 24% of Spanish words were systematically related to one another.

The second big discovery is why systematicity occurs: it’s driven by iconicity—when a word or sign resembles its meaning. For example, in English, “buzz” evokes the sound of a bee or vibration. In ASL, the sign for eat mimics the motion of bringing food to your mouth.
The researchers found that when words or signs are iconic, they’re more likely to be systematically related to one another. For instance, “sniff,” “sneeze,” and “snout” all relate to the nose and start with “sn” (a sound which is nasally resonant). In ASL, signs relating to thinking are produced near the signer’s temple.
“ASL is an ideal language for studying iconicity because it includes both highly iconic signs—like ‘eat’—and signs with little or no visual resemblance to their meaning,” says Erin Campbell, Research Assistant Professor at Boston University’s Wheelock College of Education & Human Development. “It offers a unique lens into how language might actually be more structured than we’ve traditionally believed.”
Visualizing iconicity and systematicity in sign and spoken language
To measure iconicity and systematicity, the researchers built a software system for collecting and annotating large datasets of sign language videos with the help of the Software Application & Innovation Lab (SAIL) at Hariri Institute. This enabled them to identify which signs were semantically related to one another. They combined this information with phonological information from ASL-LEX 2.0, the largest database of lexical and phonological properties of American Sign Language. This approach enabled the team to visualize the whole landscape of the language networks to describe how spoken and sign words relate to each other.
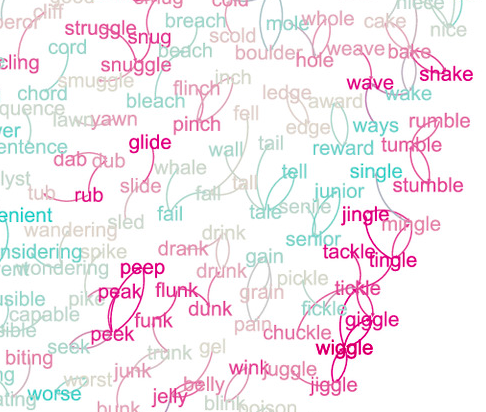
For comparison across languages and language modalities, the researchers leveraged parallel datasets of spoken English and Spanish. To explore phonological and semantic relationships between word pairs, they presented network visualizations of systematicity for each language.
While the dataset’s primary use is for researchers to understand how language structure connects to human or machine learning, it offers many promising applications says Naomi Caselli, Associate Professor of Deaf Education, Director of the Deaf Center, and Director of the AI and Education Initiative at Boston University.
“The research not only challenges fundamental language theory, but has practical applications,” says Caselli. “It could improve how we teach language to children or new learners by highlighting word patterns rather than requiring memorization. It could help adults suffering from aphasia relearn language. It opens new possibilities for advancing machine learning models, paving the way for more effective, inclusive, and intuitive language technologies.”
ASL Tests the Limits—And Expands Them
This study employs ASL as a compelling edge case for understanding human language.
“In programming courses, we teach beginners to think about the “edge cases,” rare ways an application might be used,” says Caselli. “If we don’t, then edge cases can mess up the entire application, and this is similar with language. We have to be intentional about seeking out different ways people think about language. These “edge cases” of human language can be extremely informative to make a much more robust understanding of how language works.”
Future Directions for ASL-LEX 2.0
This study took about seven years across multiple sites, and is the largest lexical dataset of a sign language in the world. The data and software used to build the datasets are freely available, and already teams from around the world, including Kenyan and German Sign Language, have begun working to replicate this dataset in their respective languages. This will allow the researchers to do cross-linguistic comparisons to see the prevalence of systematicity in other languages.
“With this large data set, we now have the opportunity to leverage advances in Natural Language Processing (NLP) tools for ASL, helping bridge the gap between signed and spoken languages,” says Caselli.
The full list of word pairs and the code used in the statistical analyses are available at https://osf.io/5y6s4/.
The study was funded by the National Science Foundation (1918252, 1625793, 2234787 to NC; 1918556, 1625954 to KE and ZS, 1918261, 1625761 to AG, and 2234786 to ZS)
Paper Citation: E.E. Campbell, Z.S. Sehyr, E. Pontecorvo, A. Cohen-Goldberg, K. Emmorey, & N. Caselli, “Iconicity as an organizing principle of the lexicon,” Proc. Natl. Acad. Sci. U.S.A. 122 (16) e2401041122, https://doi.org/10.1073/pnas.2401041122 (2025).
Study authors:
Erin Campbell, PhD, Research Assistant Professor at Wheelock College of Education & Human Development, Boston University
Zed Sehyr, PhD, Assistant Professor, Communication Sciences and Disorders, Crean College of Health and Behavioral Sciences, Chapman University
Elana Pontecorvo, EdM, PhD student, Speech, Language and Hearing Sciences, Sargent College of Health & Rehabilitation Sciences, Boston University
Ariel Cohen-Goldberg, PhD, Associate Professor of Psychology, Tufts University
Karen Emmorey, Professor Speech Language Director, Laboratory for Language & Cognitive Neuroscience, San Diego State University
Naomi Caselli, Associate Professor of Deaf Education, Director of the Deaf Center, and Director of the AI and Education Initiative, Boston University