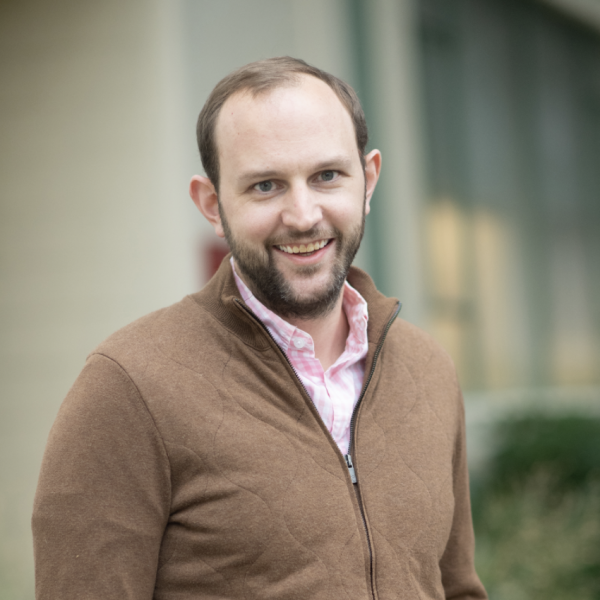
Assistant Professor
Professor Huggins is a member of the Probability and Statistics research group and of the Faculty of Computing & Data Sciences. His research centers on the development of fast, trustworthy machine learning and AI methods that balance the need for computational efficiency and the desire for statistical optimality with the inherent imperfections that come from real-world problems, large datasets, and complex models. His current applied work focuses on algorithms for efficient calibration and uncertainty quantification of large-scale ecological models and methods to enable more effective scientific discovery from high-throughput and multi-modal genomic data. For more information about Professor Huggins please see his personal webpage.