These Faculty Are Using Data Science to Help Change the World
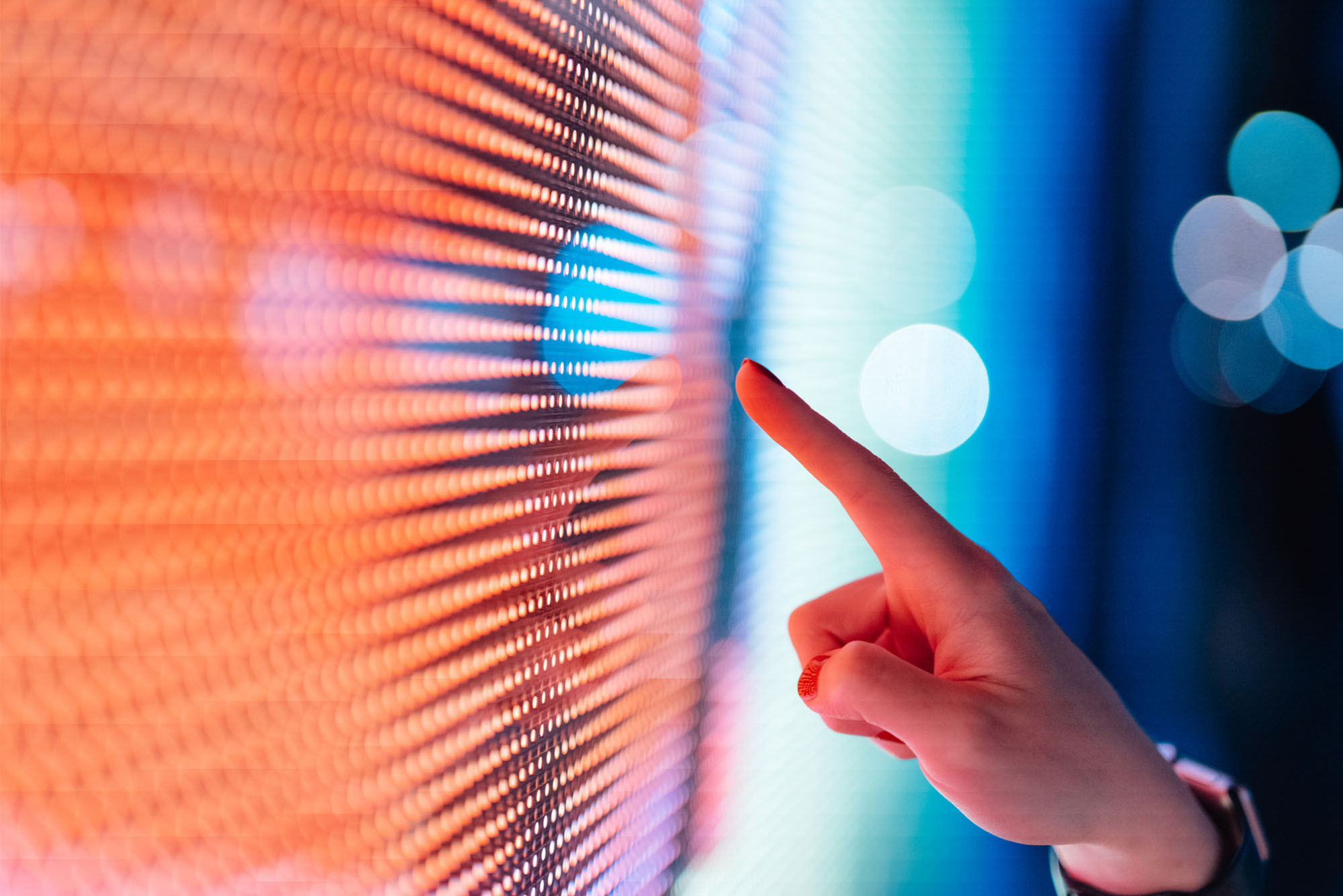
Photo by Oscar Wong/Getty Images
These Faculty Are Using Data Science to Help Change the World
Crossing disciplines and using data, they are working to predict diseases in people, to reduce the gender wage gap, to help marginalized groups like undocumented immigrants and victims of domestic violence, and more
Meet some members of the new Faculty of Computing & Data Sciences and learn how they are collaborating across disciplines and using data to predict diseases in people, to reduce the gender wage gap, to help marginalized groups like undocumented immigrants and victims of domestic violence, and more.
Brian Cleary
The intersection where computer and data sciences meet biology is where Brian Cleary lives.
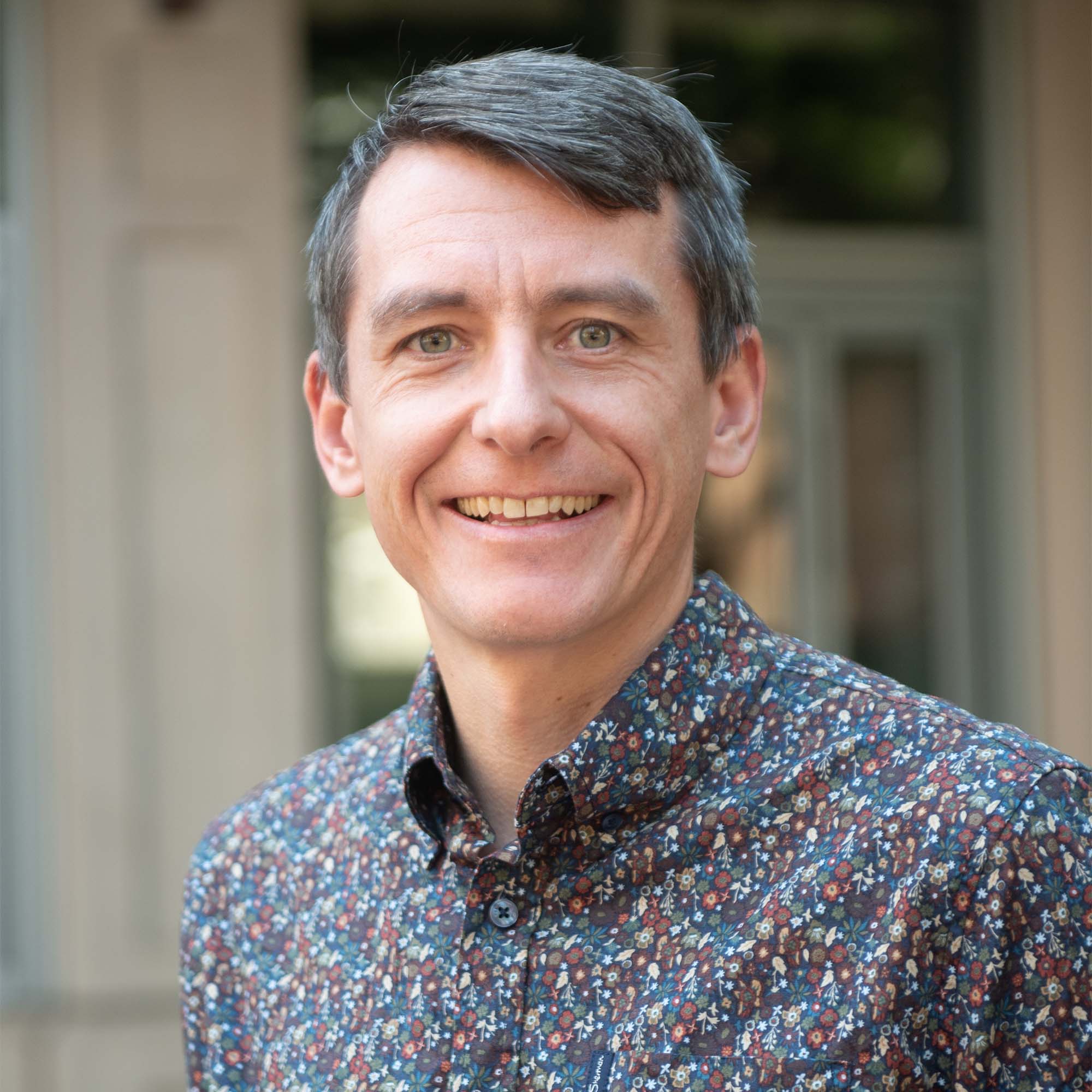
His work uses machine learning and computer science techniques to harness data that’s been accumulated over decades to study how cells and tissues in the human body work—and how genes express themselves.
Let him explain.
“So we’ll take, for example, a tissue section, and we want to know throughout the tissue, in every cell in the section that we’re imaging, how much of the gene that we are profiling is present in every single cell. Then we look for patterns in a particular cell, and we look to see which cells tend to be located near others.”
That work helps him to better understand the structure and function of the tissue, how it develops, and how diseases might progress within it. Cleary joined BU in the summer of 2022 as an assistant professor in the Faculty of Computing & Data Sciences and a core faculty member in the Bioinformatics Program.
There’s a wide range of real-world applications for Cleary’s approach, including understanding the development of organs and systems, and disease prediction.
Most recently, Cleary has been interested in brain development. But beyond the brain, the possibilities for using these techniques are endless.
“There are so many different types of applications,” Cleary says, “and that’s one of the reasons why it’s exciting for me to work in the very basic science.”
Ngozi Okidegbe
Ngozi Okidegbe has long been interested in racial justice in society. But she also has become an expert in computer coding, algorithms, and new technologies developed through big data. Those passions provided the perfect opportunity for her to become BU’s first dual-appointed faculty member in law and data sciences, and she can’t wait to get started. She is an associate professor in the School of Law and an assistant professor in the Faculty of Computing & Data Sciences.

As an example of her research and work, Okidegbe points to injustices related to pretrial release decisions within the legal system. When a person is charged with a crime, she explains, a judge has to determine whether to release or detain a person while their trial is pending. But studies have shown that class and race often affect this process, resulting in the pretrial detention of a large number of defendants, primarily Black or brown, who pose no danger or flight risk.
This disparity in who gets released pretrial is one reason why some jurisdictions are now turning to using pretrial algorithms that use big data, statistical methods, and information about a defendant to produce a prediction about whether that defendant would miss a court appearance or be arrested for a crime while awaiting their trial. The hope is that the predictions provided by the algorithms will help judges make their decisions in a less biased way.
But it’s not that simple, Okidegbe says. Algorithms for pretrial decision-making are not perfect. They are still built off of data produced by legal institutions, and therefore they tend to produce racially unequal positions about marginalized or underrepresented communities.
“Algorithms may offer a path to decreasing the harm that the criminal legal system and other legal systems enact on racially or otherwise marginalized communities,” Okidegbe says. “But unlocking this path requires us to prioritize these communities.”
Jonathan Huggins
It can be difficult for scientists, engineers, and anyone who does research or works in industry to extract reliable conclusions from large data sets.
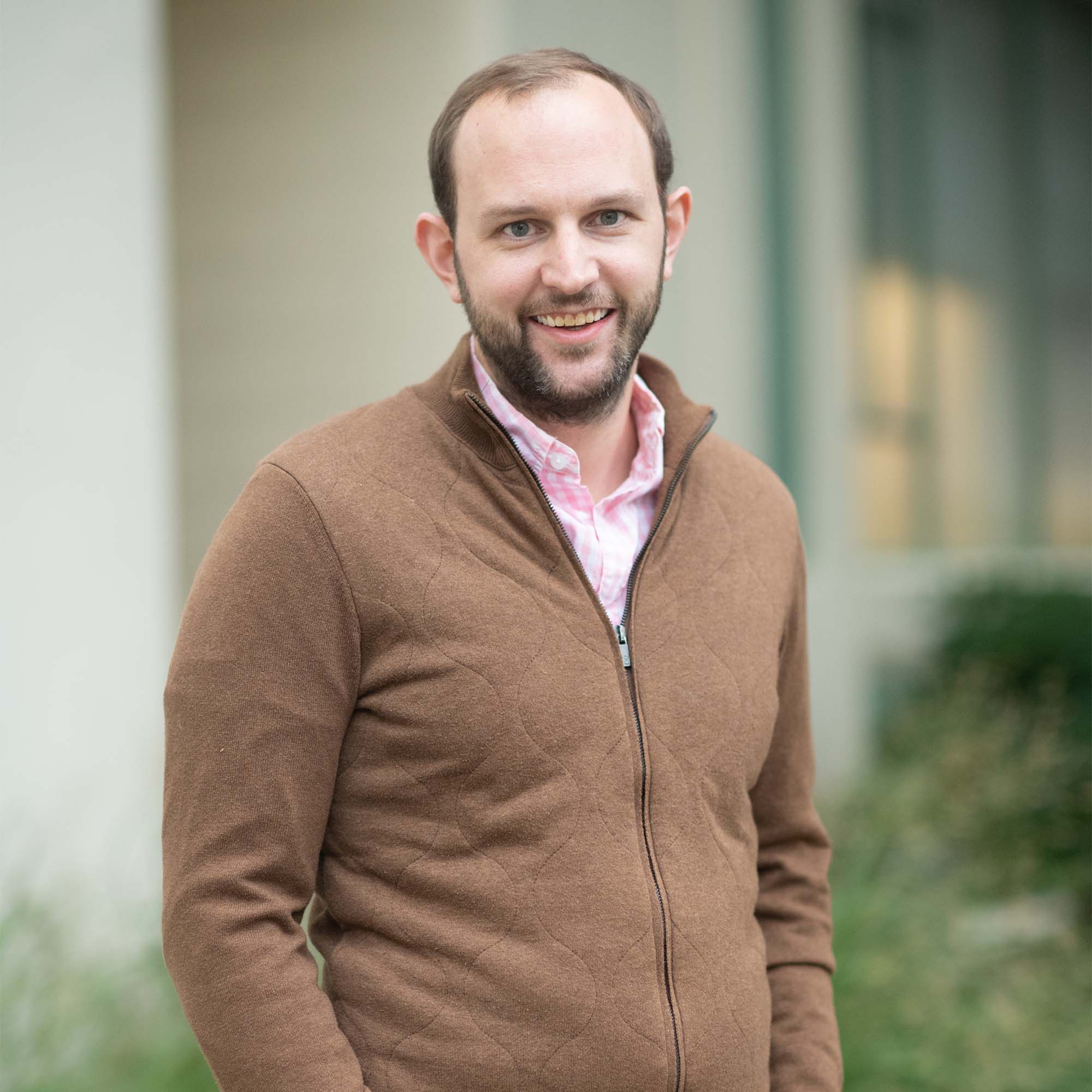
That’s why Jonathan Huggins, mathematics and statistics and Faculty of Computing & Data Sciences assistant professor, has devoted his research to finding data analysis tools that are computationally efficient. In other words, some methods aren’t guaranteed to incorporate all the available data and produce as close to a perfect result as you could want, and Huggins wants to change that.
“You’d really like to have methods that you can trust, you know are going to work, not just because they’ve worked in the past, but because you have some more kind of theoretical guarantees,” Huggins says.
There are two applications Huggins is entrenched in now. One deals with cancer genomes and creating a tumor by analyzing the data from the mutational processes—everything that can combine to give you cancer. From there, the task is to try to accurately reflect these processes to determine the cause of the cancer.
A second application is ecological forecasting: using data from the worldwide carbon cycle—how much carbon do trees and plants release, how much carbon is in the air, for example—and factoring local impacts like climate. The ultimate goal is to predict how much certain systems will be affected by climate change.
“So I have a method and I want it to be computationally efficient. I also want it to be statistically efficient, using all available information from the data that I have, so I’m not sort of wasting the data that I have available to me,” Huggins explains. “What are the trade-offs there, how do I extract everything that I can to be as efficient as possible?”
Leonidas Kontothanassis
After a decade and a half working at Google and Facebook, Leonidas Kontothanassis was contemplating retirement or a few more years working somewhere more enriching.
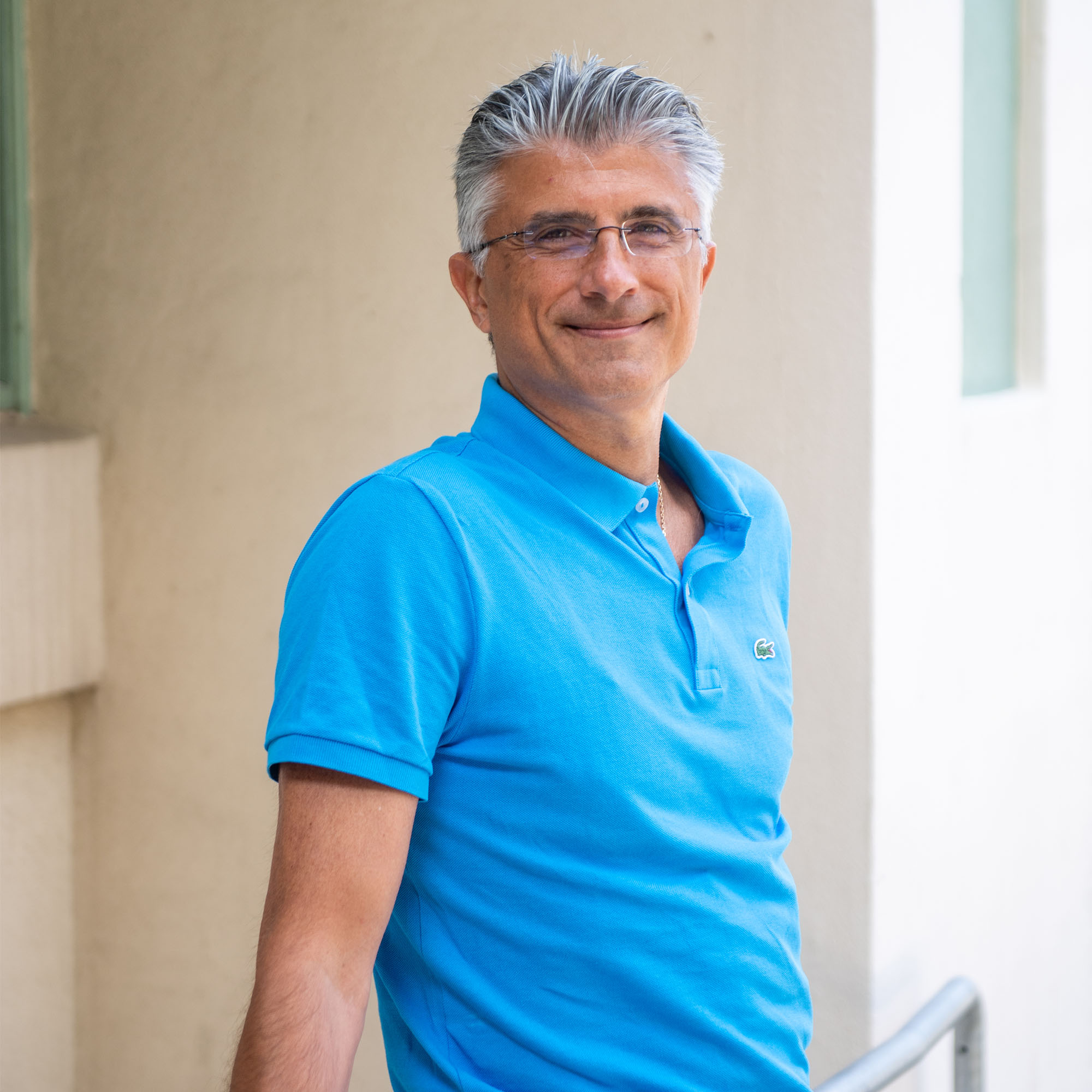
When the opportunity came his way to return to BU for the first time since 1999 (when he was an adjunct), he leapt. While in the corporate world, he’d figured out exactly what he wanted to teach students before they made their way into the real world: the skills to hit the ground running at a new job.
Mainly, Kontothanassis, who is the inaugural MassMutual Professor of the Practice in the Faculty of Computing & Data Sciences, wants CDS students to learn to operate in different cloud environments, which he says most companies, including start-ups, will be building on for the foreseeable future.
“So I know the Google system the best…I was there for 12 years,” he says. “There’s about 106 different products in the Google cloud. I’m not saying you have to know all 106, but it would be useful for people to come out and know what’s the difference between, say, Spanner and Cassandra and SQL and sort of whatever other storage product…why would you use one over another?”
He’s hoping to create a course or series of courses that familiarizes students with the various cloud environments, so that when they come out and they go to work in the industry, they’re better prepared for whatever comes their way.
His corporate experience has taught Kontothanassis that it can sometimes take new hires fresh out of college six months to a year to learn a company’s internal systems and software engineering practices. Once students are able to take the type of course Kontothanassis wants to provide them, that onboarding time will shrink, which benefits the employer and employee.
Life at a couple of the world’s largest tech companies had its perks, but now Kontothanassis is looking forward to a different type of satisfaction.
“I think this is something that’s going to be more rewarding than the previous work that I’ve been doing,” he says.
Allison McDonald
If you didn’t know that signing up for something as simple as electricity for your home could wind up putting your data in the hands of a government agency, you should know that Allison McDonald is working to make sure there’s more transparency in data sharing.
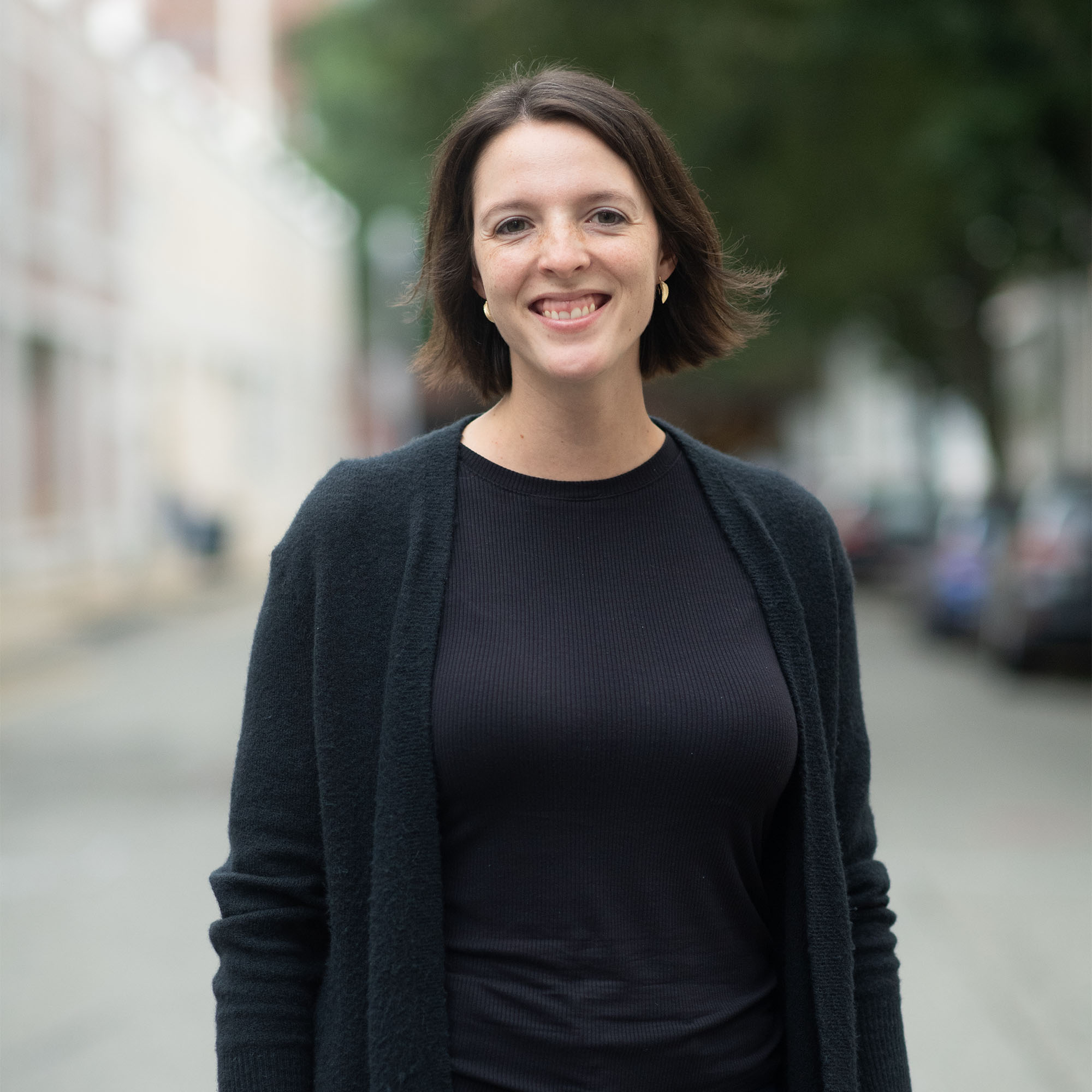
McDonald’s work also tries to make sure that marginalized groups—especially undocumented immigrants, sex workers, victims of intimate-partner violence—are better protected online and have better access to the internet. Sometimes these groups wind up being surveilled. Or they can find their access to certain web services being blocked based on user agreements and where they or the service is based.
McDonald, a new Faculty of Computing & Data Sciences assistant professor, wants the internet to be safer and more equitable for all.
“Some of the goal of doing this sort of quantification component is to more effectively build tools for policymakers and for technologists to address these discrepancies,” McDonald says, “and giving, especially to policymakers and lawmakers, tools to argue for a more equitable way to regulate the internet.”
McDonald is also using internet measurement techniques to see how widespread these problems are and how far her solutions have to reach. She’s excited to be able to use her computer science background in a meaningful manner.
“I felt that I really had to do the work to find a path that was focused on social good,” she says. “Concentrating on privacy and security was one of those pathways that felt like it was focused on making people safer and reducing harm.
“The ability to help people and understand ways the status quo is harmful and finding ways to imagine and build better futures is what really got me to stay on this path.”
Yannis Paschalidis
Yannis Paschalidis does the type of data science research that ties into health, science, engineering, biology—and the list just keeps on going. His work reflects just how much data science is ingrained into virtually every field.
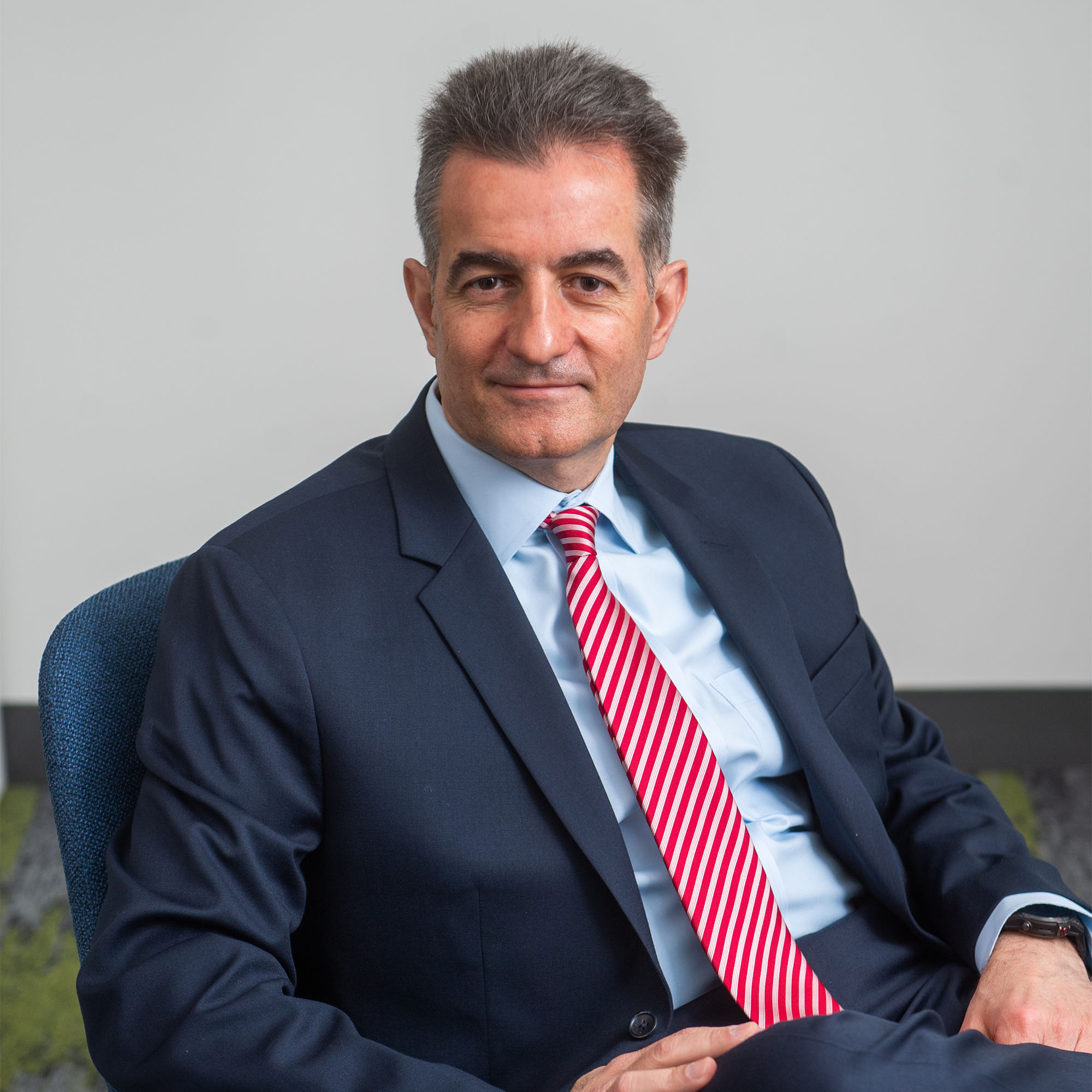
“I would say that from the methodological point of view, there is a link to all of this, developing new methods. Some of these methods have their basis in optimization, and optimization is one of my specialties,” Paschalidis says. In addition to being the director of BU’s Rafik B. Hariri Institute for Computing and Computational Science & Engineering, he is also a Faculty of Computing & Data Sciences founding professor and a professor of engineering.
Paschalidis’ knack for optimization has led to developing algorithms that will allow the next generation of autonomous systems to be able to operate in an unstructured environment. Instead of a self-driving car, for example, learning to ride on the highway, it can be taught to navigate open terrain where it might encounter surprising obstacles.
Paschalidis also works with sustainability in energy companies to more efficiently have demand meet supply rather than vice versa.
And his work in healthcare leverages large amounts of data so that physicians can make better decisions and predictions about patients and devise their daily treatment plans.
And then there are also computational biology questions Paschalidis wants to answer about protein interactions and cell structures.
If there is one tie that runs through all of his various efforts with data, it’s the reward he receives from it. “I think from a motivational point of view, doing work in healthcare, doing work in biology, is obviously extremely motivating because you can save lives. Not at the level that the physician would do,” Paschalidis says, “but at the level that designing and developing systems would help physicians save lives.”
Kate Saenko
Kate Saenko’s work focuses on training artificial intelligence.

The goal is to make AI better at recognizing images and texts, classifying and recognizing objects and activities in images and videos, and doing textual analysis of images and videos related to texts such as closed captioning.
It’s a natural career path for someone who always had a proclivity for advances in technology that once seemed far off into the future and are now part of our everyday lives.
“Well, I think I’ve always been interested in science fiction,” says Saenko, a computer science associate professor and a Faculty of Computing & Data Sciences founding member. “And so when I was choosing what to study in graduate school, I was attracted to the idea that we can have computers recognize human speech and talk to people like people. So it seemed very ‘science fictiony’ to me at the time.”
Of course, nowadays, she says, science fiction has become reality. But the link between the two is what attracted her to the general field of AI and speech recognition. She hopes her work will help advance AI to places previously only seen in the movies or in novels.
“We want to create algorithms or computer methods that mimic the way that humans do tasks—that’s the goal of artificial intelligence,” she says. “And part of that is the human ability to do pattern recognition and understand complex signals like images, for example, and be able to very quickly categorize them into different categories or describe them in language, which is another way of categorizing complex signals into distinct categories.
“The high-level goal is trying to somehow replicate human intelligence.”
Adam Smith
Adam Smith is a professor of computer science and a Faculty of Computing & Data Sciences founding member. But he soon found himself infatuated with the concept of differential privacy, or the way data collectors can share information about the data while at the same time withholding information about the individuals in the data set.
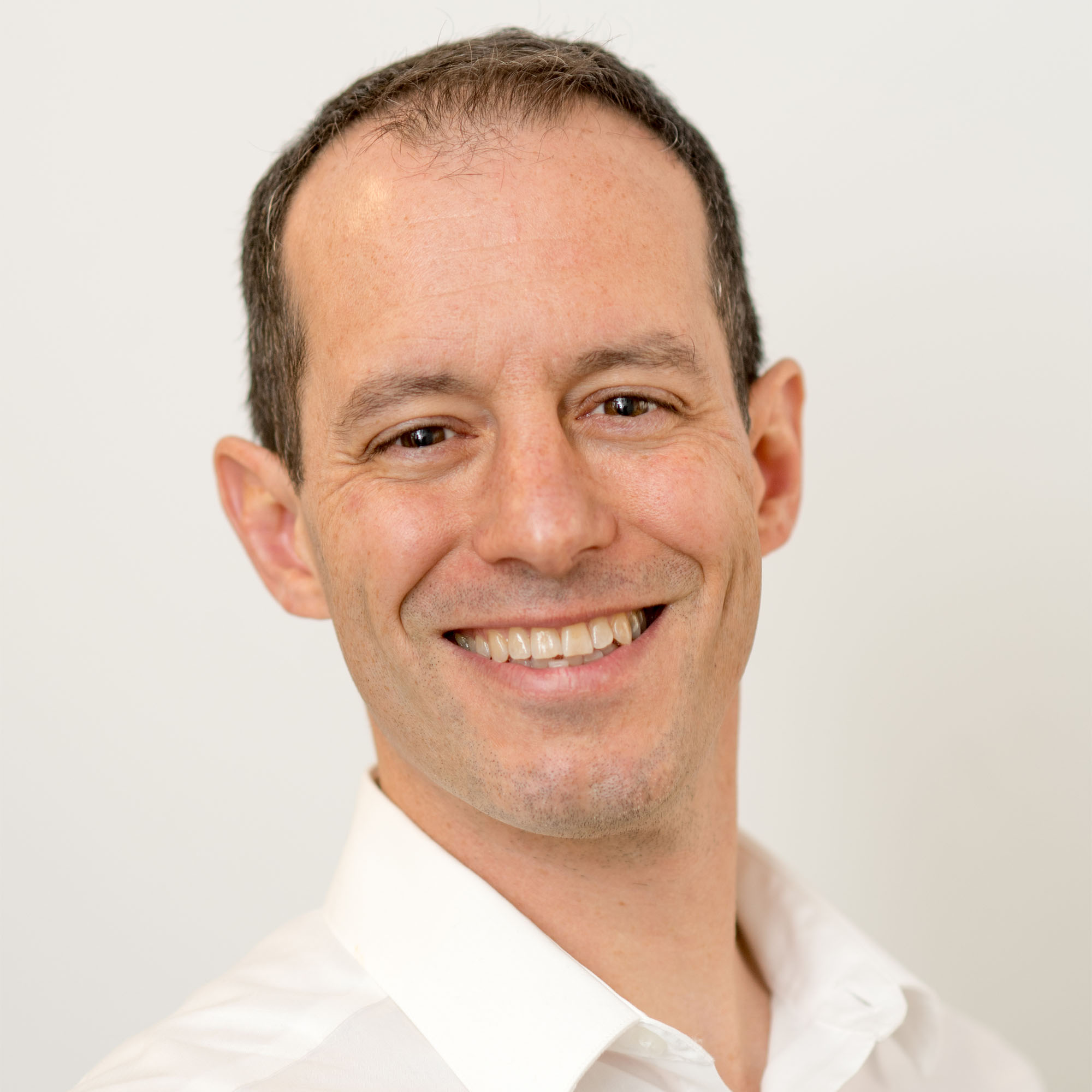
Hospitals want to aggregate data to tell each other about interesting statistics and trends. The US Census Bureau wants to paint a rich picture of the demographics of the country. But when it comes to releasing their data, these organizations, and others, always wind up leaking at least a small amount of sensitive information.
“And so this leads to this basic conundrum of trying to understand how can you release as much as possible without ending up releasing the raw data. Which in many of these data sets is very sensitive,” Smith says.
“So what I’ve been interested in is where that line is and how to formalize it.”
That’s where differential algorithms come in.
“I’ve done lots of work on algorithms that satisfy these constraints so that they don’t leak too much about any one individual,” he says, “for useful statistical processing of data, training machine learning models, doing statistical analysis, basically doing just the kind of things people want to do with these data.”
It’s important to make the algorithms more efficient to hinder someone from taking, for example, the census data and reverse engineering it to find the individual data. This same approach helps hospitals protect sensitive information. And it even aids tech companies like Google when it’s programming machine learning tools to provide users with word predictions.
Data science, he says, is about “trying to kind of take the revolutions we’ve seen in data collection and processing and computation and make them as widely useful and available as possible.”
Mayank Varia
Using data without actually seeing the data. That’s the fresh approach Mayank Varia takes with cryptography—and with data science.
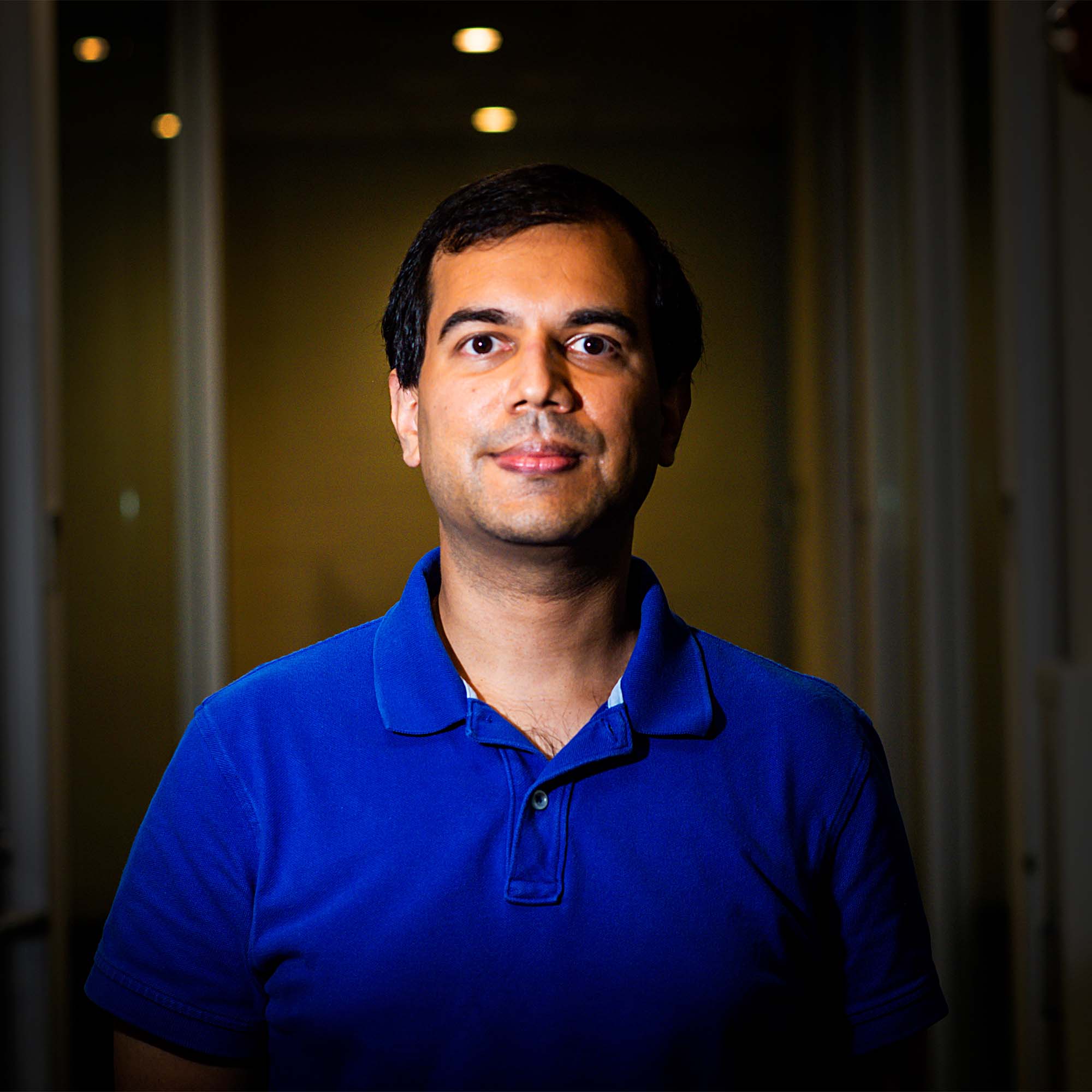
“I think of it as social science that masquerades as mathematical science,” says Varia, a Faculty of Computing & Data Sciences associate professor and codirector of the Center for Reliable Information Systems & Cyber Security (RISCS). “We’re using tools and principles from math—but for the purposes of achieving socially desirable outcomes, especially in the digital world.”
In order to achieve those desirable outcomes, Varia and some colleagues have had to build off the idea of “secure multiparty computation,” which allows parties to share data to accomplish a goal, while not sharing more than they want to with one another because their data might reveal information they need to protect.
“The goal is the computing of data without seeing it,” Varia says.
“So basically it’s about helping people to get data science done where they don’t fully trust each other to do data sharing and they don’t fully distrust each other,” he explains. In other words, they have an interest in doing data science together, just warily.
This approach to data science has helped the City of Boston and the Boston Women’s Workforce Council reduce the gender wage gap. By using a data aggregation algorithm, employers are able to share salary information—without revealing the actual salaries of their employees.
Varia and others have also used cryptography to help the Greater Boston Chamber of Commerce’s Pacesetters Program determine how often large companies subcontract work to minority-owned businesses. Another application has been used to link criminal records and identify repeat perpetrators of sexual assault.
There’ll be more world-improving applications in the future, all while Varia continues toward his original goal back when he first shifted from mathematics to data science.
“It’s about how can we harness data, potentially very sensitive data, but still harness its abilities to help with accountability, oversight, and transparency of the metrics that we think are useful in moving society forward,” Varia says.
Comments & Discussion
Boston University moderates comments to facilitate an informed, substantive, civil conversation. Abusive, profane, self-promotional, misleading, incoherent or off-topic comments will be rejected. Moderators are staffed during regular business hours (EST) and can only accept comments written in English. Statistics or facts must include a citation or a link to the citation.