The Machines Are Coming

*In order to highlight the rapid emergence of artificial intelligence—and to reflect the uncertainty and indeed ominousness felt by many observers of AI’s rise—we created all images (unless otherwise noted) using DALL-E, a deep-learning model developed by OpenAI to generate digital images from natural language descriptions. After generating the images using keyword prompts from the text of the story and additional descriptions such as “futuristic” or “steampunk,” a graphic designer then modified the images digitally into their final form.
The Machines Are Coming
BU Wheelock faculty see promise (and a little peril) in the rapid expansion of artificial intelligence

lgorithm-informed machines learn what we like to buy and look at on the internet, feeding us more of that. Smart “assistants” greet us on our favorite websites to answer questions and point us in the right direction. Robots assemble almost everything we own, from our cars to our televisions to our clothing. Artificial intelligence (AI) can quickly scan enormous bodies of information, like the internet, and provide comprehensive answers. But there are risks and potential abuses that stem from AI, from the ChatGPT-written college admission essay to the replacement of certain human professions by machines. BU Wheelock faculty certainly have those reservations. But mostly, they are optimistic—or realistic, perhaps—about the ways educators may soon use AI to provide even more personalized and comprehensive supports to students. BU Wheelock asked a few of them to share, in their own words, the uses they see for AI in their respective areas of study, with a warning or two about the technology’s limitations.
USING AI IN DEAF EDUCATION
NAOMI CASELLI, ASSISTANT PROFESSOR OF DEAF EDUCATION AND CODIRECTOR OF THE BU AI & EDUCATION INITIATIVE

Work on sign languages has lagged behind spoken languages in part because we haven’t had the tools to measure sign language production. AI may be able to help. Our understanding of how people produce and perceive speech has benefited from a host of tools for automatically transcribing speech, identifying which speech sounds are being produced, and taking detailed measurements of the quality of speech sounds and the position of the tongue and mouth.
New advances in computer vision are making it possible for us to take similar measurements of signs. We have begun to utilize human pose estimation—a computer vision-based technology that identifies and tracks specific points on the human body—to locate the hands and fingers as a signer produces a sign, which we have used to teach a computer how to recognize what a person is signing. While I worry about the ethical implications of language technologies—like pressure to replace rather than augment human teachers and interpreters—I am eager to see progress on AI-powered sign language computing help to make language technology accessible to sign language users.
AI and Language Education
Ashley Moore, Assistant Professor of Language & Literacy Education
I’m feeling an equal mix of hope and concern. On one hand, colleagues at BU have used ChatGPT to quickly create first drafts of reading texts at the appropriate level for their students. Learners who lack a conversation partner have exploited ChatGPT as a functional—yet still rather unexciting—target language partner. Similarly, a learner who’s unable to afford a personal language tutor can use a generative AI tool to suggest edits on an early draft of their written work and hopefully learn from those changes.
But because AI tools draw from huge amounts of existing data, they tend to reproduce dominant linguistic practices, threatening linguistic diversity and exacerbating linguistic discrimination. For example, we’ve spent decades trying to undo the myth that it’s necessary or even desirable for learners to mimic the language practices of so-called native speakers. However, some companies are already marketing AI chatbots that “mimic the culture, behavior, and gestures of native English speakers” in “authentic” conversations. Similarly, just as some users of global English varieties are successfully asserting the equal legitimacy of their English, the use of AI as a proofreader threatens to flatten that linguistic diversity into standardized forms of English associated with white, middle-class native speakers from countries like the United States.
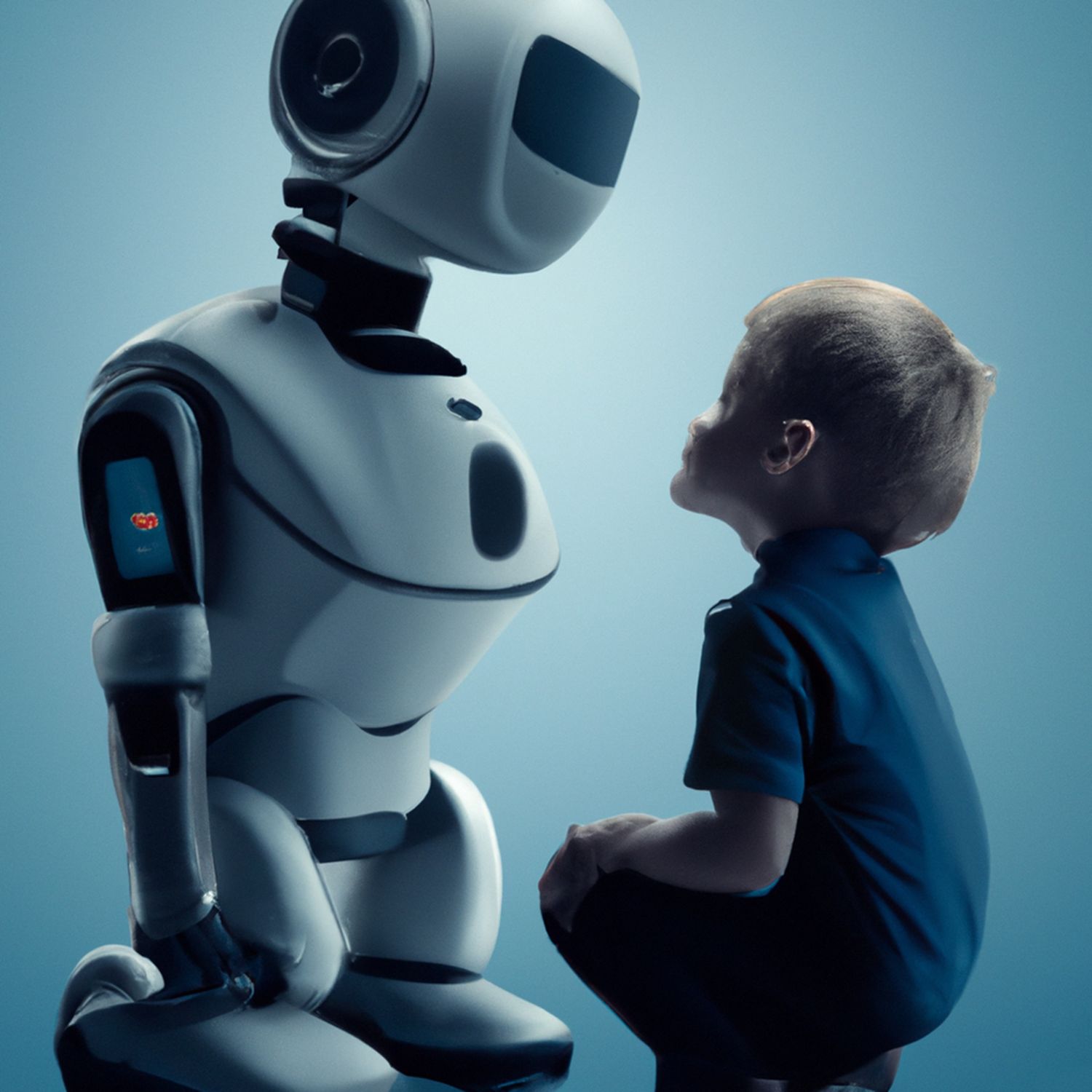
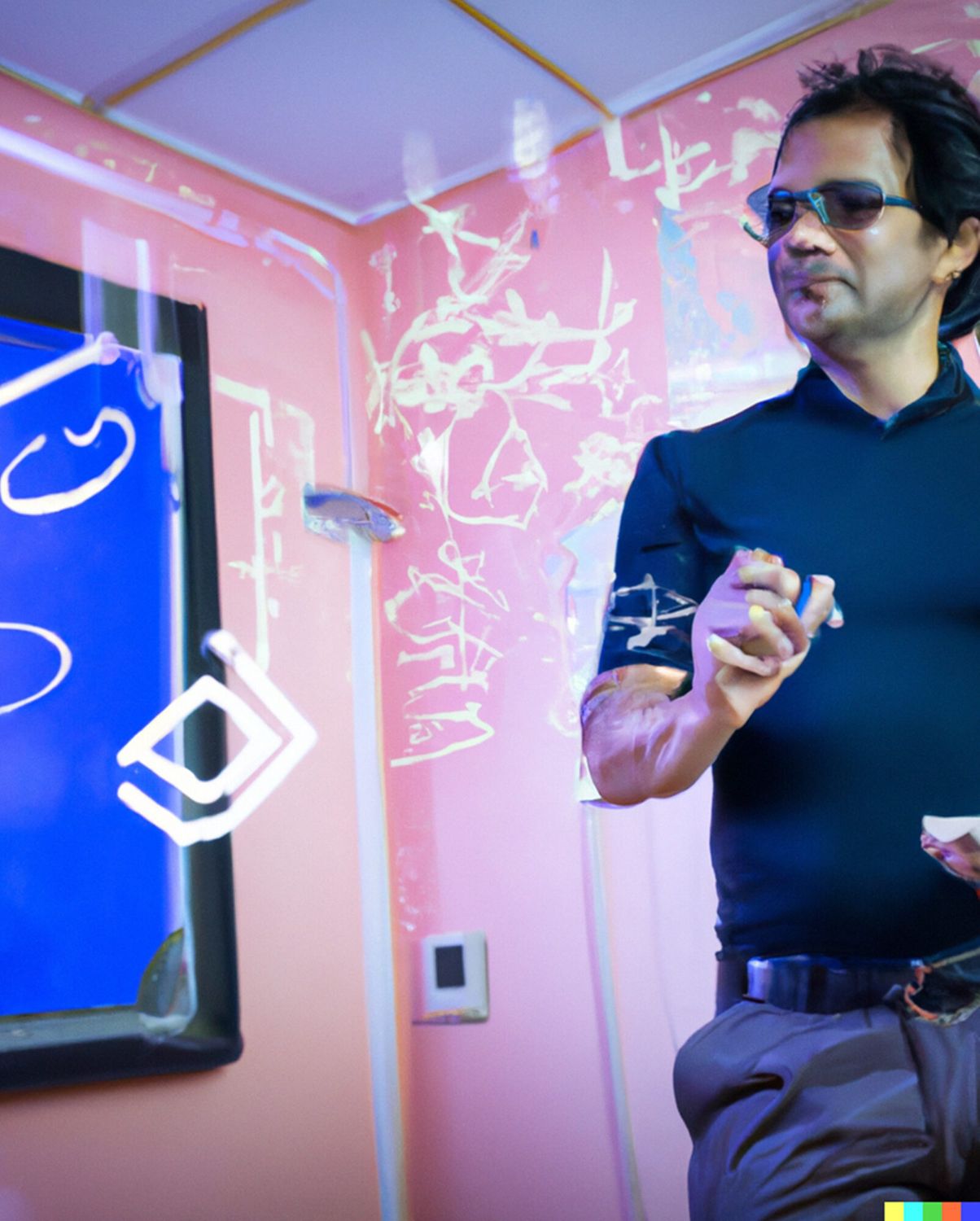
Evaluating Internet Credibility With AI
Elena Forzani, Assistant Professor of Literacy Education
AI changes the practice of evaluating credibility on the internet positively and negatively. First, the negative: tools like ChatGPT don’t necessarily write credible, representative texts by gathering information from diverse sources and representing marginalized voices. In constructing texts, ChatGPT can produce, or reproduce, inaccurate, biased information. Thus, AI can actually create an echo chamber of misinformation, perpetuating inaccurate ideas.
When evaluating text, we need to first determine whether an AI tool helped construct it, which can be hard to do. Disclaimers on AI-generated or AI-assisted content may help us do this in the future, though this practice is not widely used yet. We also need to build into digital literacy education how AI tools construct information, which requires the evaluator to have an added layer of knowledge. Additionally, the credibility of a piece of information can depend on who is asking about it and how well the information applies to that person. AI tools such as ChatGPT do not differentiate well between users. Users therefore still need to evaluate credibility for themselves, which ChatGPT acknowledges at the beginning of many of its responses.
On the positive side, when evaluating information on a new topic, AI can help us quickly conduct a background search to determine the different arguments and perspectives, who is representing them, and why. Given that AI cannot evaluate credibility well, this may be one of its best uses in helping humans evaluate text. In developing interventions to teach students information evaluation, we are beginning to build both of these implications into instruction.
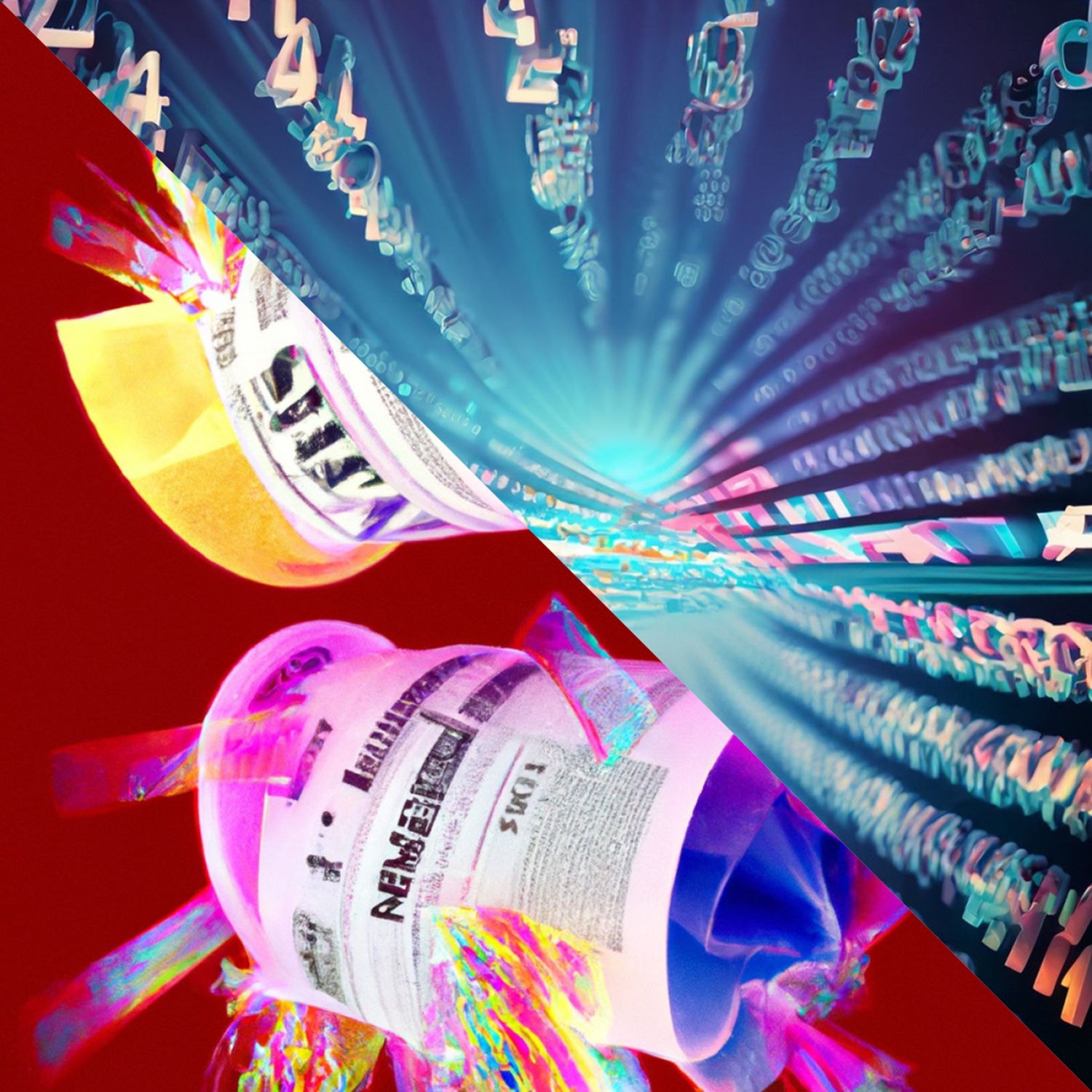
Can AI Predict Learning Disabilities in Students?
Hank Fien, Nancy H. Roberts Professor of Educational Innovation, Director of the Wheelock Institute for the Science of Education, and Director of the National Center on Improving Literacy
I am working with three colleagues—Ola Ozernov-Palchik, BU Wheelock senior research scientist; Eshed Ohn-Bar, BU College of Engineering assistant professor; and Will Tomlinson, director of the Software & Application Innovation Lab at the Rafik B. Hariri Institute for Computing and Computational Science & Engineering—in a partnership with the open-source software company Red Hat to build an open education-data platform. It will be able to ingest large, multimodal data sets—including behavioral and learning data, audio and video data, and brain imaging data—and apply AI and machine learning models to better predict and identify children who may be at risk for learning difficulties or disabilities earlier and more accurately than current methods. The platform can also help us identify children who may respond better to individualized, intensive supports than standard evidence-based interventions. We envision providing these open tools and technologies to any researchers or educational districts that have current data sets.
Our second goal is to develop an intelligent tutoring platform that can provide direct and personalized learning supports to students, enable adaptive and interactive experiences, and address and correct any learning misconceptions. Meanwhile, an intelligent tutor could provide insights to teachers and enhance the ability of educators to better differentiate reading instruction for children with and at risk for learning disabilities. Imagine an intelligent teaching buddy in a teacher’s ear offering tips to improve their students’ learning, in real time.
The expansion of AI will require educational training for teachers, learners, and parents. So, while we are working to develop new tools and technologies, we also need to develop education and workforce training tools to properly utilize AI in classrooms and communities.
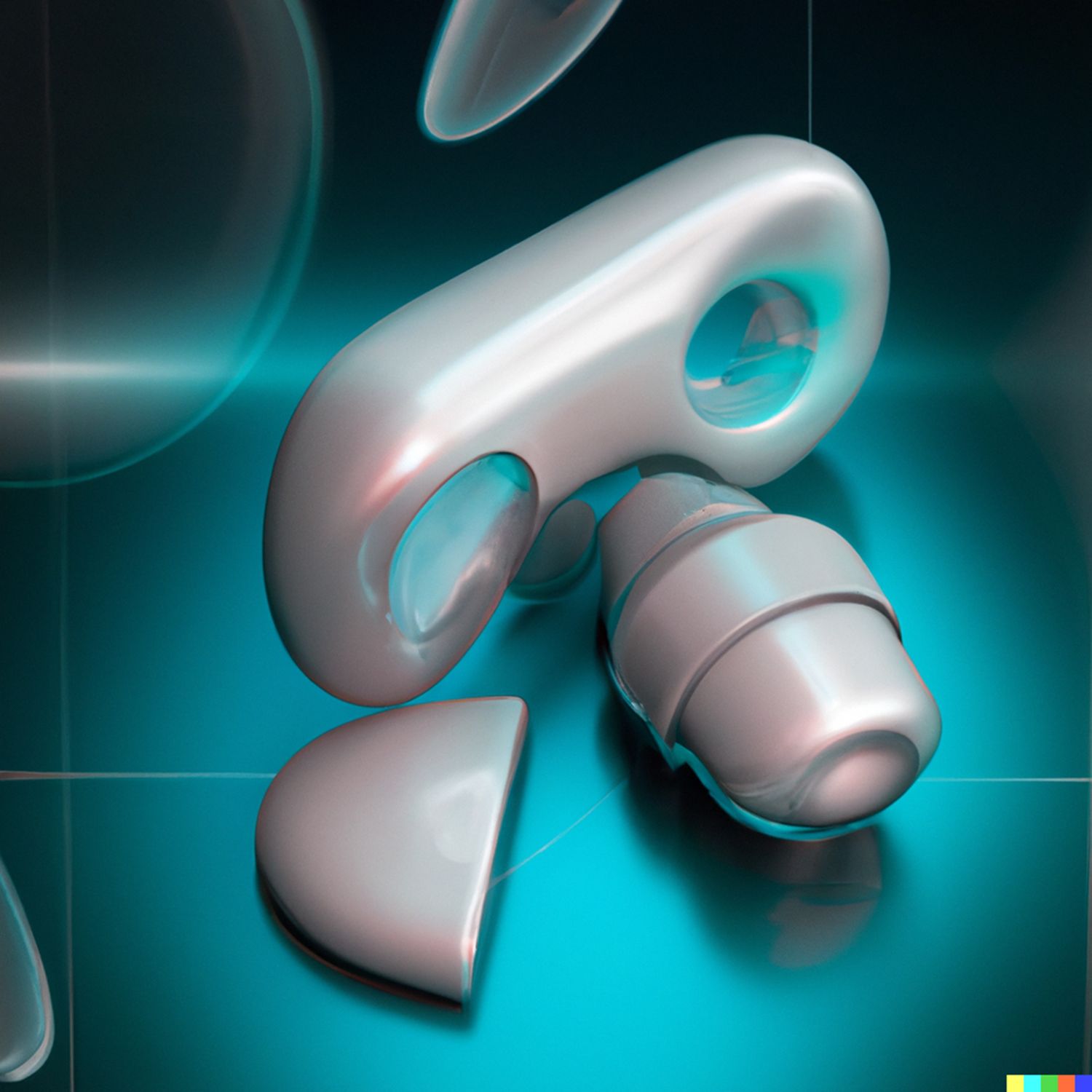
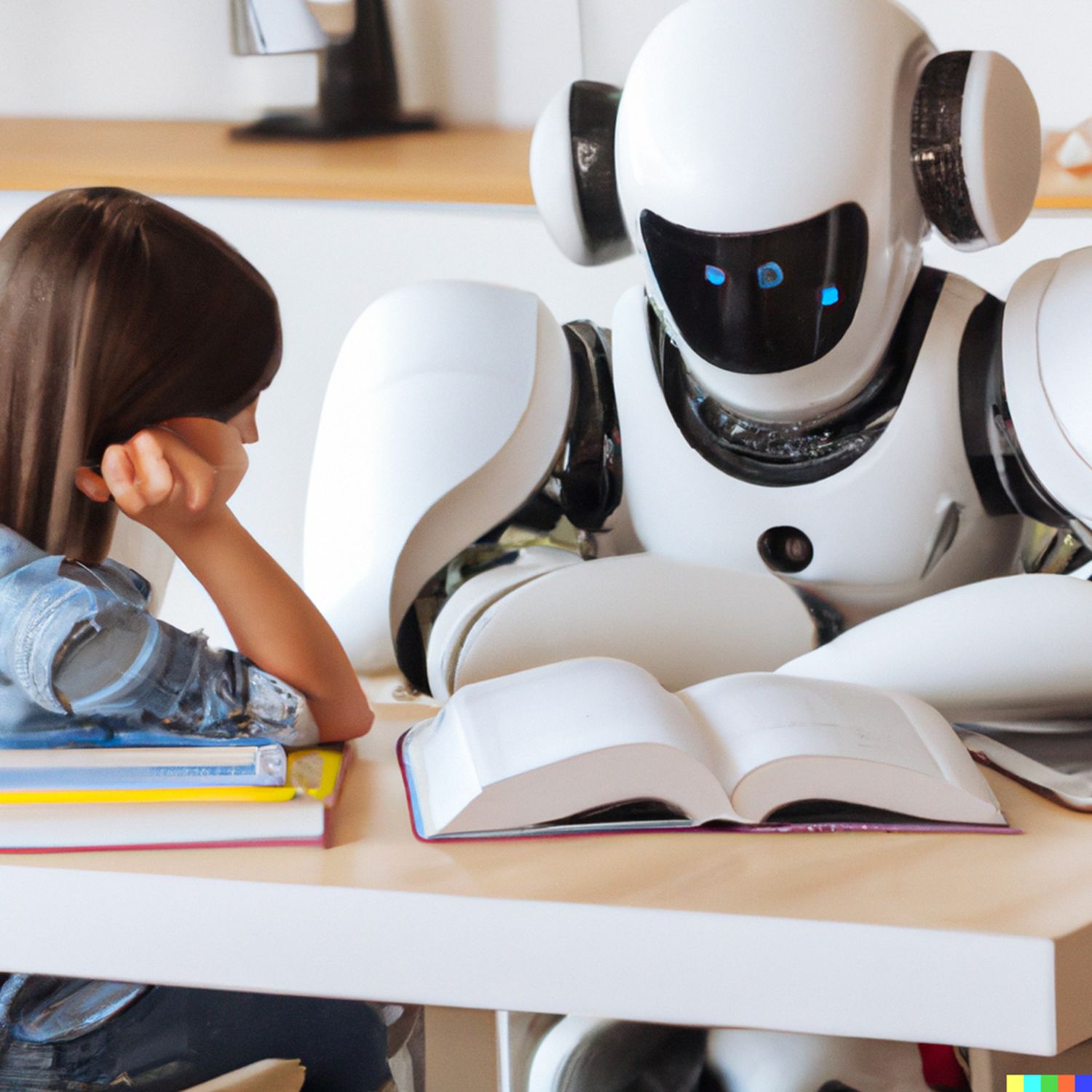

Using AI to Predict College and Career Readiness
V. Scott H. Solberg, Professor of Counseling Psychology & Applied Human Development
Pandemic-related learning loss and disengagement among high school and college students amplified concerns that this generation of youth will not be ready to maximize their potential in today’s world of work. Nationally, only 60 percent of high school graduates enter a two- or four-year postsecondary program; only 36 percent and 58 percent graduate, respectively, our research has shown. We’ve also found that nearly 12 percent of young adults aged 19 to 27 are disconnected from work and education. Those working full-time average less than $13 per hour, with less than 35 percent earning more than their state’s “living wage” benchmark.
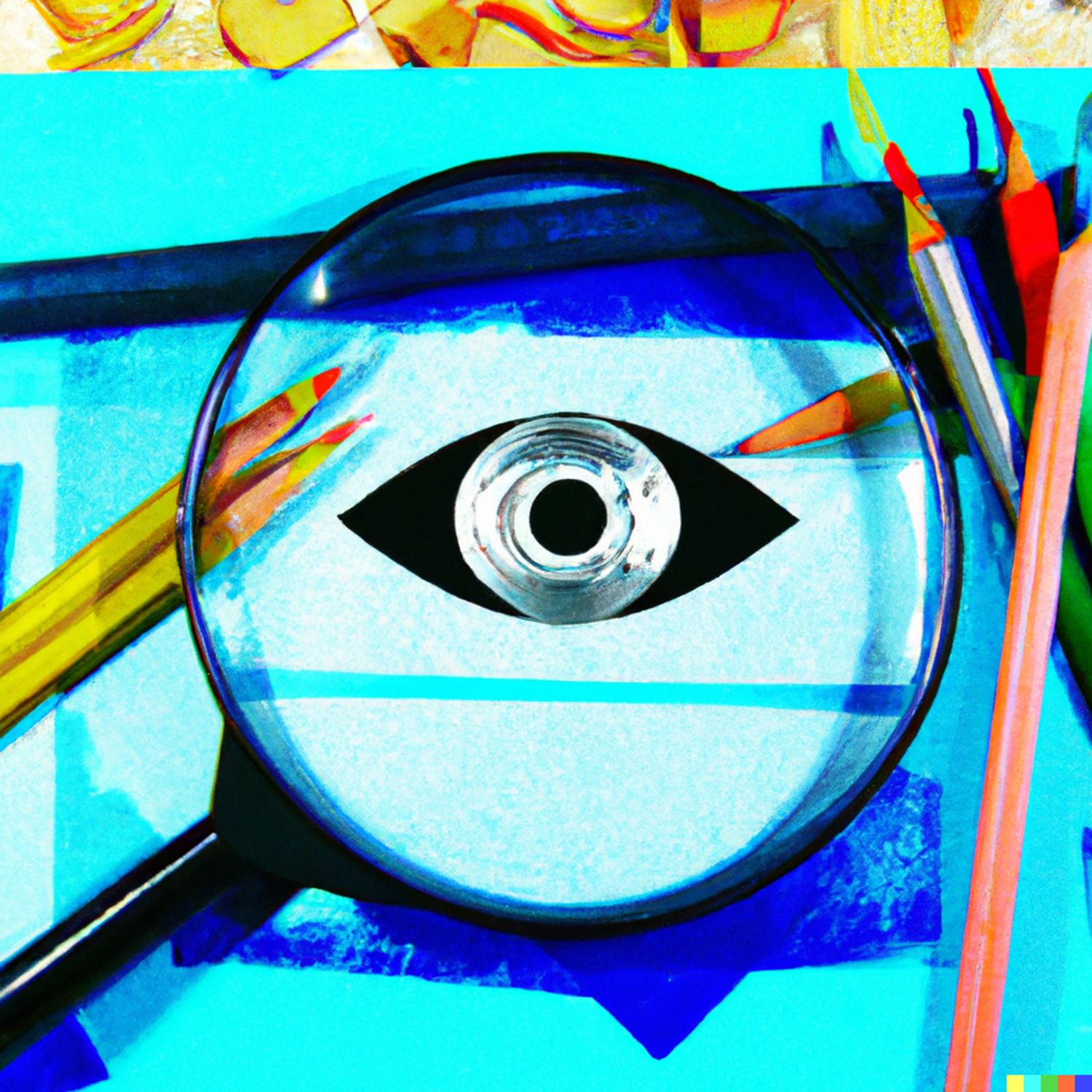

In collaboration with colleagues in BU’s College of Arts & Sciences—Derry Wijaya, an assistant professor of computer science; Sarah Bargal, a research assistant professor of computer science; and Chenwei Cui (GRS’23)—I am working to create a reliable and scalable assessment tool that alerts educators and colleges whether and to what extent students are setting high future aspirations and proactively engaged in striving to realize those aspirations. Using career narratives collected from 1,000 high school students, we are studying whether large language models such as BERT, PaLM, LLaMA, and GPT that now achieve state-of-the-art performance on many benchmark natural language processing tasks can achieve reliable results in predicting career readiness levels among high school youth. This solution has major implications as an assessment tool to monitor individual youth progress through secondary education, as well as a tool postsecondary
institutions can use to assess applicants.
Assessing Teacher Quality with AI
Nathan Jones, Associate Professor of Special Education and founding member of BU’s Faculty of Computing & Data Sciences
My research centers on how we think about and identify effective teaching among practicing teachers and how we maximize learning experiences among preservice teachers.
One area where I see promise in AI is in increasing the frequency and quality of feedback we’re able to provide beginning educators. For the past several years, I’ve been leading a National Science Foundation–funded project to improve preservice general educators’ ability to use instructional practices known to support students with disabilities in mathematics. As part of this project, we have developed an innovative set of performance tasks, where candidates respond to brief instructional scenarios in an internet-based environment. These performance tasks give researchers information on whether candidates have developed the skills we’ve been teaching them.
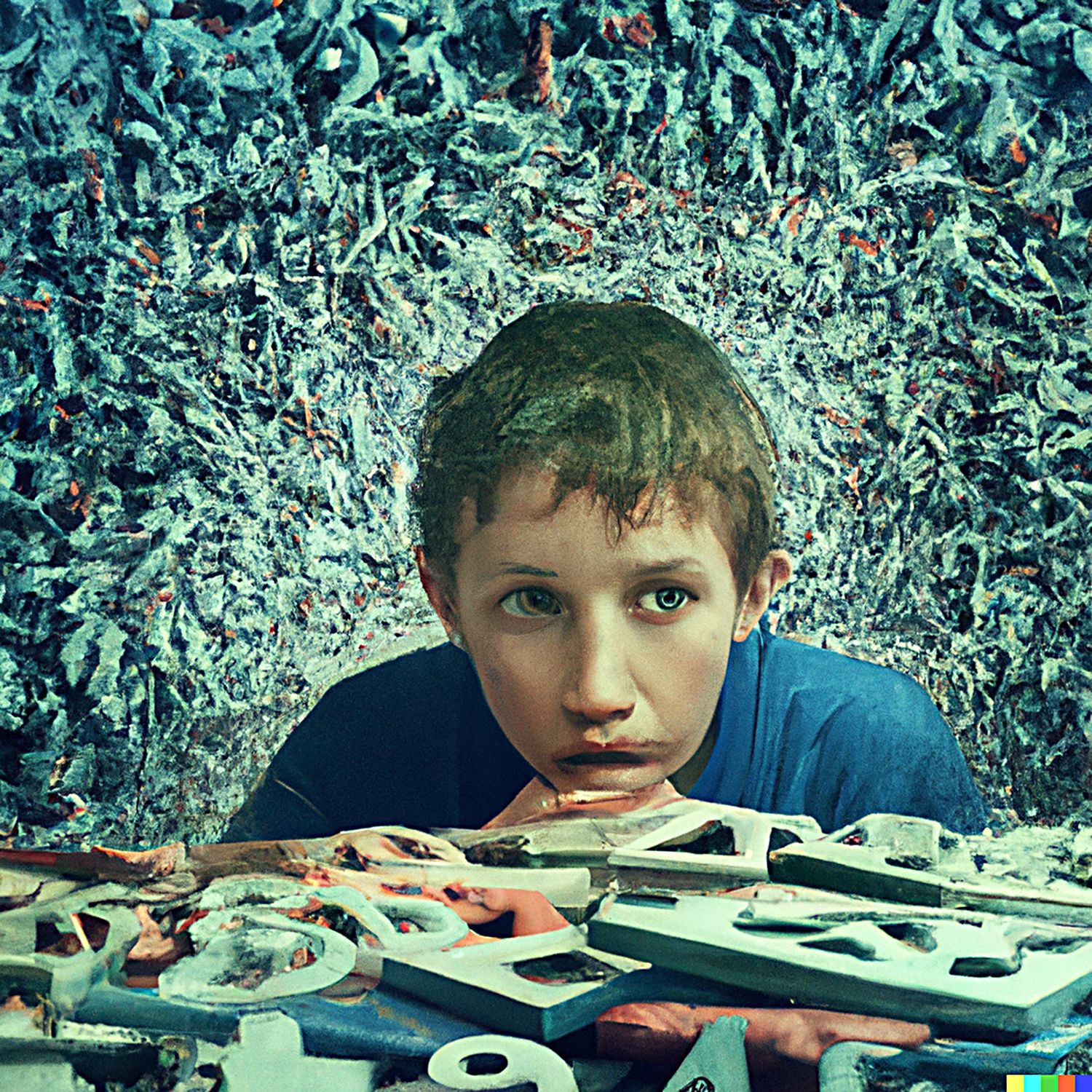

For teacher candidates, it’s an opportunity to practice these skills in a low-stakes context. Ideally, it would be great to be able to give immediate, targeted feedback to candidates so that they could improve more quickly. But scoring any sort of measure based on observation takes time, and usually, it takes considerable training to ensure that scores accurately and appropriately capture a teacher’s performance. Our team has been working with an expert in data science methods, Jing Liu at the University of Maryland, to create automated scoring and feedback. This approach, using natural language processing to examine transcripts from performance task videos, is meant to speed up the cycle of practice and feedback. Our hope is to leverage machine learning to reduce the scoring burden on teacher educators and researchers, ideally improving the scalability of our training tools. There are a number of key issues we are still puzzling over: Can automated scores approach the quality of human scores? Do these automated approaches introduce bias? As we work on the lingering questions, however, we remain optimistic about AI’s long-term contributions to teacher preparation.
Comments & Discussion
Boston University moderates comments to facilitate an informed, substantive, civil conversation. Abusive, profane, self-promotional, misleading, incoherent or off-topic comments will be rejected. Moderators are staffed during regular business hours (EST) and can only accept comments written in English. Statistics or facts must include a citation or a link to the citation.